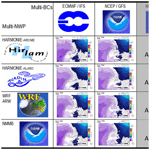
The AEMET-γSREPS over the Antarctic Peninsula and the impact of kilometric-resolution EPS on logistic activities on the continent
Alfons Callado
Mauricia Martínez
Benito Elvira
Kilometric-resolution Ensemble Prediction Systems (EPSs) will be the new state-of-the-art forecasting tools for short-range prediction in the following decade. Their value will be even greater in Antarctica due to the increasingly demanding weather forecasts for logistic services. During the 2018–2019 austral summer (1 December–31 March), coinciding with the Southern Hemisphere Special Observation Period of the Year of Polar Prediction (YOPP), the 2.5 km AEMET-γSREPS was operationally integrated over the Antarctic Peninsula. In particular, the Antarctic version of γSREPS comes up with crossing four non-hydrostatic convection-permitting NWP models at 2.5 km with three global NWP driving models as boundary conditions. The γSREPS forecasting system has been validated in comparison with ECMWF EPS. It is concluded that γSREPS has an added value to ECMWF EPS due to both its higher resolution and its multi-boundary conditions and multi-NWP model approach. γSREPS performance has a positive impact on logistic activities at research stations and its design may contribute to polar prediction research.
- Article
(1662 KB) - Full-text XML
-
Supplement
(797 KB) - BibTeX
- EndNote
Logistic actors play a decisive role in Antarctica. They provide essential services to research community such as flights, vessels, and small boats in order to distribute scientists on the field. Furthermore, they offer residence to the scientists in Antarctic stations or camp sites, and sometimes even help scientists with their equipment. Most of their activities depend on skillful predictions (Bromwich et al., 2020; Jung et al., 2016; Powers et al., 2003), which are crucial, especially during medical emergencies (Monaghan et al., 2003; Powers et al., 2012). Those services are often provided by national meteorological services. Particularly, the Spanish Meteorological Agency (AEMET) has been commissioned to provide weather forecasts for the Spanish stations in Antarctica.
Antarctic Peninsula, where the Spanish Juan Carlos I (JCI) research station is located, is strongly influenced by the Antarctic circumpolar trough and the quasi-stationary Amundsen–Bellingshausen Seas Low (Gonzalez et al., 2018; Hosking et al., 2013). It generates during summer, secondary lows and sometimes mesoscale cyclones, which usually cross northwest to southeast the region. Moreover, the complex orography of the island where the station is located, strongly modifies the usually stable low-level flows. As a consequence of these local scale interactions, weather conditions may differ substantially from one side of the island to the other. This is a great challenge for the weather forecasters who provide meteorological information to different users at different places. In the recent years, scientists at JCI has been increasingly adventuring further from the main station in order to expand their research. This conflicts with the growing concern about the risks involved in increased human activities in Antarctica (Dawson et al., 2017). In order to ensure the safety and security of the scientific expeditions, and to maximize their schedule, more demanding, specific and precise weather forecasts are requested. However, the low density of weather observations assimilated in most of the Southern Hemisphere reduces the performance of the numerical weather prediction (Bromwich et al., 2020; Jung et al., 2016).
To fulfill this requirement, during the Spanish Antarctic campaign 2018–2019, AEMET tested and used operationally the recently developed high-resolution Ensemble Prediction System (EPS) AEMET-γSREPS (hereafter γSREPS; Fig. 1). γSREPS improves the confidence in the forecast by giving an uncertainty to the short-term forecasts. In this article, we present and describe the Antarctic version of γSREPS that is, as far as the authors know, the first convection-permitting LAM-EPS that operationally run at the continent. Other operational EPS run over the continent. The AMPS ensemble is widely used in Antarctica. It has 15 members and runs at 24 and 8 km resolution in a domain that extends to the entire continent (Bromwich and Powers, personal communication, 2019; freely accessible to real-time forecasts trough https://www2.mmm.ucar.edu/rt/amps/, last access: 19 September 2020). Unlike AMPS, γSREPS has a higher resolution but its domain is limited to the Antarctic Peninsula and Drake Passage. Here, we evaluate the available probabilistic forecasting tools of the Spanish forecasters during the campaign 2018–2019, i.e ECMWF EPS and γSREPS. We show an objective validation of the ensembles and one case study where γSREPS successfully helped the weather forecaster on duty in the decision-making process.
The AEMET-γSREPS (Frogner et al., 2019; Quintero Plaza and García-Moya Zapata, 2019; Santos, 2018) is a multi-boundary conditions (BC) and multi-model (NWP) kilometric resolution LAM-EPS, which currently runs over the Iberian Peninsula, Canary islands and the Antarctic Peninsula. In the Antarctic version, the integration area is centered on Livingston Island (Fig. 2) with 565×469 grid points in a lambert-conformal conical projection. It has a horizontal resolution of 2.5 km and 65 vertical levels. The multi-boundaries approach deals with the initial and lateral boundary sources of uncertainty taking the boundary conditions (BC) from three Meteorological Centers that execute global NWP models (centre/NWP): ECMWF/IFS, NOAA-NCEP/GFS and Canadian CMC/GEM. The BC are taken from global deterministic models integrated 12 h before γSREPS cycle. That means that the 12 h global forecast are used as initial conditions in γSREPS. Multi-model technique addresses the model errors and uncertainties executing 4 different LAM-NWP models (centre or consortium/NWP): HIRLAM/HARMONIE-AROME, ALADIN/ALARO, NOAA-NCAR/WRF-ARW and NOAA-NCEP/NMMB (Fig. 1). As a result of using each of the 3 BCs to integrate each one of the 4 mesoscale LAM-NWP models, γSREPS is constituted by 12 members (Fig. 1). The main technical features of γSREPS, the 3 global driver NWP models and the 4 regional NWP models are described in Table 1. Notice that overall settings of γSREPS NWP models and their parametrizations have not changed with respect the 20 members over the version that runs over the Iberian Peninsula. Nonetheless, it is believed that the impact of these general settings is limited during the summer season at the Antarctic Peninsula when temperatures are mild. Indeed, settings of the γSREPS NWP models are similar to those used in the North Hemisphere in high latitudes, e.g. HARMONIE over the Scandinavian countries.
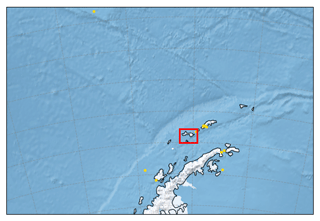
Figure 2Full area of simulation of the Antarctic version of γSREPS showing the 12 surface observation stations (yellow points) used for the objective verification. Red rectangle shows the domain plotted in Fig. 5.
Table 1Some main features and some references of γSREPS, the 3 Global driver NWP models and the 4 LAM-NWP models. Ver is the NWP model version, hr is the approximate horizontal resolution, vlev is the vertical resolution, ass is assimilation technique, prj is the projection, ts the time step in seconds. Note that in global models we indicate the resolution used.
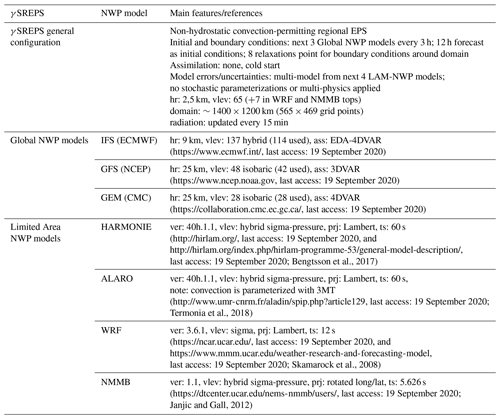
The 2018–2019 Spanish Antarctic campaign took place from 15 December until 31 April coinciding with the Southern Hemisphere Special Observation Period (SH-SOP) of the Year of Polar Prediction (YOPP) (Bromwich et al., 2020). Throughout most of this period, the γSREPS Antarctic version was integrated at 00:00 UTC up to 48 h in a domain showed in Fig. 2. Qualitative and quantitative verification and case studies over the Iberia Peninsula (not shown) indicate that, in general, multi-model γSREPS performs better than other ensemble designs such as the multi-physics EPSs and the unimodel LAM-EPS using SPPT stochastic parametrizations. Compared to them, γSREPS shows higher consistency, reliability, spread-skill ratios and threshold skills. However, the variable meteorological conditions over Antarctica may have distinct impacts on the different short-range EPS designs that should be investigated.
Forecast verification is essential for monitoring model performance and eventually improving the accuracy of the model (Ebert et al., 2013). A verification was performed during the period comprised between 1 January to 28 March 2019. We compared the 00:00 UTC cycles of the two EPS used by the weather forecasters during the campaign: the global 50-member ECMWF EPS (16 km horizontal resolution) with the regional 12-members γSREPS (2.5 km horizontal resolution). For this purpose, we used the synoptic surface observations at 00:00 and 12:00 UTC of 12 stations retrieved from ECMWF MARS (Meteorological Archival and Retrieval System) (Fig. 2), totalizing 950 observations for each lead time. One of the challenges of objective verification over the Antarctic Peninsula is the few numbers of available observations which are clustered around the international Antarctic stations and do not cover the Southern Ocean.
The verification was performed using the HIRLAM-ALADIN R package (harp) for verification (available at https://github.com/andrew-MET, last access: 19 September 2020). A summary of the verification of the more significant parameters verified is shown in Table 2. In this article we discuss the 2 m temperature (T2m), 10 m wind speed (S10m) and visibility (vis) (Fig. 3). The last two variables are particularly important to consider for the groundwork activities at the research stations. However, the verification scores for other variables can be consulted in the Supplement. Figure 3 shows the spread-skill relationships, the rank histograms at the forecast time H+36 and the continuous ranked probability score (CRPS). The spread and skill inform about EPS consistency: the closer the spread of the ensemble forecast and the error in the ensemble mean (the skill), the better. The rank histogram provides information about the distribution of the ensemble forecast showing biases, lack of variability or overspread in the ensembles (Hamill, 2001): the flatter the histogram, the better. The CRPS express the distance between the probabilistic forecast and the value observed (Hersbach, 2000): the closer to zero the better.
Table 2Verification scores summary comparing γSREPS versus ECMWF EPS. Color key: blue, γSREPS quite better than ECMWF EPS; light blue, slightly better; white, similar performance; grey, ECMWF EPS quite better than γSREPS and light grey, slightly better. The parameters verified are mean sea level pressure (Pmsl), 2 m temperature (T2m), 2 m specific humidity (Q2m), 10 m wind speed (S10m), visibility (Vis) and total cloud cover (CCtot). The verification scores are spread-skill ratio, Continuous Ranked Probability Score (CRPS), Rank Histogram, BIAS, Reliability, Relative Operating Characteristic (ROC) and its area (ROCA), Brier Score (BS) and Skill Brier Score (BSS) and relative (or economic) values. The leadtimes used for parameters are: 12, 24, 36 and 48 h. The thresholds for parameters where used to elaborate the last 4 scores of the table are: −5, 0, 5 and 10 ∘C for T2m; 10, 20, 40, 60, 90 and 120 km h−1 for S10m; 200, 1000, 5000 and 9999 m for Vis.
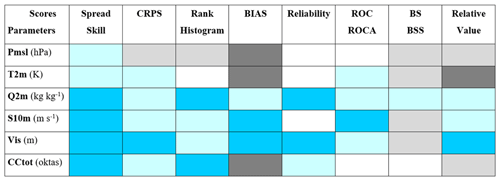
The temperature at 2 m is a basic surface verification variable but not very important for safety and security at the summer season stations over the Antarctic Peninsula. In this season, temperatures are mild at South Shetland Islands. γSREPS probabilistic T2m forecast skill in terms of CRPS is slightly worse than ECMWF EPS (Fig. 3c). This is produced by a cold bias observed in γSREPS (Table 2, Fig. 3b). The cold bias is easily explained because 9 of the 12 synoptic stations in γSREPS are over the sea and not over the land meanwhile in the ECMWF EPS all these grid points have some fraction of land. If the land-sea mask of the EPSs were considered, only 3 stations remain in both EPS strictly over land to do the verification. In consequence, it was decided not to apply masks keeping 12 stations. Anyway, the γSREPS T2m spread-skill ratio is better than ECMWF EPS (Table 2) due to a significant increase in spread although the skill is worse penalized by the sea cold bias. In fact, ECMWF EPS is under-dispersive and does not manage to represent the observation extremes.
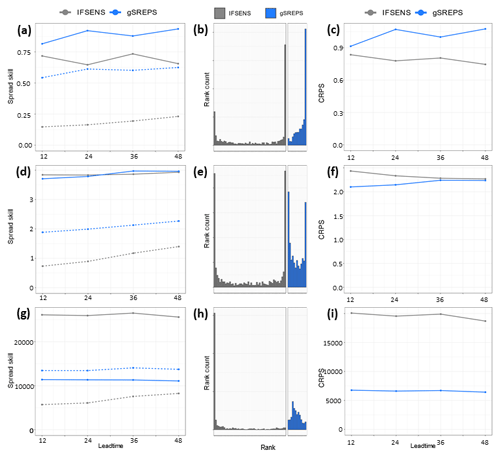
Figure 3Surface objective verification of γSREPS system compared with ECMWF IPS for (a, b, c) 2 m temperature, (d, e, f) 10 m wind speed and (g, h, i) visibility. The scores shown are (a, d, g) spread-skill relationship [the continuous line indicates the RMSE and the dashed line indicates the Spread], (b, e, h) rank histograms at 36 h forecast and continuous rank probability score (c,f,i).
The wind speed is one of the most important variables for station and groundwork operations, which depends strongly on its intensity. Forecast wind speeds shows in both EPSs an underdispersive behavior in rank histograms (Fig. 3e). It is more pronounced in ECMWF EPS (Fig. 3d) meaning that γSREPS misses less strong wind events. The smoothed relief of the ECMWF EPS with 18 km of horizontal resolution implies less influence of orographic winds. Skill is similar in both EPS, but slightly better for γSREPS as it could be interpreted from CRPS (Fig. 3f) and ensemble mean RMSE (Fig. 3d).
Another critical parameter for groundwork operations, especially on the glacier, is visibility. Reduced visibility and whiteout prevent a clear view of the crevasses and it is a hazard to the scientists that work on the glacier. γSREPS provides a great improvement for visibility with respect ECMWF EPS with a significant better CRPS (Fig. 3i) and spread-skill relationship (Fig. 3g). The latter with a remarkable reduction of the ensemble mean RMSE. Nonetheless γSREPS is overdispersive (Fig. 3h, g). This excessive spread is probably a consequence of the use of 4 different diagnostics of visibility in each one of the 4 LAM-NWP. In spite of the γSREPS overdispersion, the over-forecast of low visibility cases is clearly an improvement for forecasters with respect to ECMWF EPS where they are entirely missed.
Overall γSREPS verifies better than ECMWF EPS (Table 2) adding value to the forecasts as subjectively highlighted by forecasters. The added value is especially observed for those important variables for safety and security in Antarctic operations. This indicates a potential added value of γSREPS over global EPSs, as showed and discussed by Frogner et al. (2019) for HarmEPS. Some specific conclusions from Table 2 are: the spread-skill ratio of γSREPS is better than ECMWF EPS due to its increase on spread, Brier Score and Bias are worse for γSREPS which could be related to double penalty issue for its higher horizontal resolution (2,5 km instead of 18 km), but CRPS indicates that the overall probability forecast is better and ROC/ROCA shows the γSREPS potential improvement without bias which could be achieved through calibration of parameters. The main limitation for ECMWF EPS looks to be its underdispersion meaning that it does not manage to represent the observation extremes. The higher resolution of γSREPS is probably the main key for its better performance, but it could be argued that its multi-boundary and multi-NWP model approaches play a role as well, dealing better with synoptic and mesoscale uncertainties.
Apart from objective validation, it is necessary to collect a compilation of case studies in order to subjectively evaluate the operational use of the models. There are multiple examples of case studies of high impact weather at populated areas of the world, but there are little focused on Antarctica. Maybe the most paradigmatic case study made in the continent is the analysis of Monaghan et al., (2003), who evaluated the performance of different forecasting model systems in the rescue of Dr. Ronald Shemenski from South Pole in 2001. Here, we show the performance and operational use of γSREPS during a low-level clouds event on 5 March 2019. Low-level clouds can interfere with the normal developing of the activities over the Hurd Glacier (e.g. Osmanoglu et al., 2014; Recio-Blitz et al., 2018) next to the Spanish Antarctic station Juan Carlos I (JCI) since they are associated with poor visibility that can blur the crevasses on the glacier. Therefore, an accurate prediction of the presence or absence of this kind of clouds is critical for the station activities.
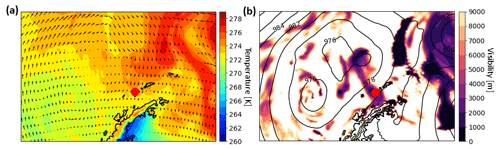
Figure 4(a) 925 hPa wind and temperature and, (b) mean sea level pressure and surface visibility simulated by ECMWF IFS on 5 March 2019 00:00 UTC+15:00. The red circle indicates the position of the Hurd Glacier.
4.1 Synoptic setting and forecasting problem
The synoptic conditions on 5 March 2019 were characterized by cyclonic conditions at the northern tip of the Antarctic Peninsula (Fig. 4). This structure is similar to the Low over the Drake Passage pattern in Gonzalez et al. (2018). The position of the low west to the South Shetland Islands produced a warm and moist advection to the area of study (Fig. 4a). This favored low-level clouds and fog over the area (Fig. 4b) which may restrict the visibility on the Hurd Glacier (next to JCI station) where some scientists develop their activities. The main challenge was to forecast whether the northern glacier would block the low-level stratus or these clouds had been able to overrun it and affect the Hurd Glacier with a pronounced visibility reduction.
4.2 Mesoscale effects and the added value of γSREPS
Figure 5 shows a comparison between the two EPSs used by the weather forecasters during the campaign. Figure 5a and b show the probability of visibility below 5000 m forecasted by both EPS at 5 March 2019 00:00 UTC+15:00. Although both EPSs show a north-to-south visibility gradient, it is less pronounced for the ECMWF EPS compared with γSREPS. Indeed, ECMWF EPS indicates a probability of 70 %–80 % of visibility below 5000 m at the northern side of the island, and around 60 % over the Hurd Glacier. In contrast, γSREPS shows probability values over 90 % at the northern side of the island, and much lower values of 10 %–20 % over the Hurd Glacier.
In Fig. 5c and d are represented the probability of ceiling, which is the height of the base of the lowest layer of clouds that covers more than 4 octas, below than 1000 ft (305 m). In this case the maximum probability values of the ECMWF EPS are located over the island, showing probability values of 80 %–90 % over the Hurd Glacier. ECMWF EPS might be simulating a cap cloud over the terrain due to the model orography is not high enough to block the clouds on the northern side. In contrast, γSREPS shows a blocking pattern in the northern side, forecasting less than 10 % probability of ceiling below 1000 ft over the Hurd Glacier.
These differences evidence the better representation of the orography in the γSREPS system with 2.5 km horizontal resolution. γSREPS was able to properly simulate the lee-side reduction of low-level clouds and increase of visibility and was instrumental for the forecaster guidance. The presence of Tangra Mountains (1700 m maximum height) at the eastern side of the island and the difference between the output of the two EPSs suggested that blocking effects were important on that day. Considering the better representation of the orography on γSREPS, the weather forecaster considered that conditions would be good enough to develop scientific activities in the area.
4.3 Impact of the forecast on operations
Using the guidance of AEMET-γSREPS, the weather forecaster on duty suggested to carry out the research activities over the glacier which was observed to be appropriate. Finally, mountain waves developed over the Southern Bay and Hurd Peninsula, leeside of the northern arm of the Livingston Island (Fig. 6). Clouds and precipitation likely accumulated in the northern parts of the island. In this case study clearly the higher resolution of γSREPS, with better orographic representation of the mountainous islands and terrains of the Antarctic Peninsula, was a key factor and an added value with respect ECMWF EPS.
This article describes the forecast performance of the operational LAM-EPS AEMET-γSREPS, used in Antarctica during the 2018–2019 summer season. The period coincided with the Year of Polar Prediction, which promotes the improvement in the prediction capabilities for the polar regions (Bromwich et al., 2020; Jung et al., 2016). During the campaign the EPS used 12 members, constituted by 3 different boundary conditions and 4 different mesoscale LAM-NWP models. γSREPS improves subjectively (case studies) and objectively (verification) the forecast skill for all the different variables studied, except sea level pressure, with respect ECMWF IFS EPS. It also increases the generally low spread of ECMWF EPS. Its multi-boundaries and multi-model design contributes, at least in part, to the increased spread. However, spread is excessive with respect to the visibility, yielding sometimes to over-predict low-visibility events. It has been observed that kilometric-resolution models have a positive impact on forecast skill in Antarctica, specially where the flow is influenced by the orography (Bromwich et al., 2005). Similarly, kilometric-resolution EPSs increase the ability to make detailed predictions and represent local uncertainties in the short-range forecasts, which have a large impact in the decision-making process for logistic and management of Antarctic activities. Last but not least, the multi-BC and multi-NWP configuration of γSREPS is suitable to study the source of the uncertainties of polar prediction in the Antarctic region, allowing the possibility to evaluate the outputs segmented by BC (synoptic and α-mesoscale uncertainties) and NWP (β-mesoscale uncertainties) similarly to Bromwich et al. (2013). Currently, γSREPS is running during the austral summer Spanish Antarctic Campaigns (1 December–31 March). It is expected to continue for next campaigns, maybe increasing the number of members in order to improve the forecasts and consequently making JCI activities safer.
The output files of γSREPS during the SH-SOP of the YOPP has been stored by the authors. Due to the large amount of data, it is not possible to publish the output files. Nevertheless, they are available upon request.
The supplement related to this article is available online at: https://doi.org/10.5194/asr-17-209-2020-supplement.
SG and AC designed this research and wrote the first draft contributing almost equally to this study. AC designed the AEMET-γSREPS and adapted it for Antarctica. AC and MM performed the objective validation. SG and BE performed the case study. All the authors reviewed the document. SG edit the final document.
The authors declare that they have no conflict of interest.
This article is part of the special issue “19th EMS Annual Meeting: European Conference for Applied Meteorology and Climatology 2019”. It is a result of the EMS Annual Meeting: European Conference for Applied Meteorology and Climatology 2019, Lyngby, Denmark, 9–13 September 2019.
The authors acknowledge the help of Pau Escribà, David Quintero and David Gil (AEMET, Predictability-γSREPS group), Andrew Singleton (MetNO, HARP), Roberto Ribas (ECMWF, BUFR), Ulf Andrae (SHMI, scripts to retrieve ECMWF EPS data), Alberto Martínez (AEMET), the Antarctic Group of AEMET and the logistic support in Antarctica of the UTM (CSIC). We also thank the two anonymous reviewers for their suggestions and comments, which significantly improved the final version of this manuscript. This is a contribution to the Year of Polar Prediction (YOPP), a flagship activity of the Polar Prediction Project (PPP), initiated by the World Weather Research Programme (WWRP) of the World Meteorological Organization (WMO).
This research has been supported by the Departament d'Innovació, Universitats i Empresa, Generalitat de Catalunya (grant no. 2017 SGR 1102). Activities of the AEMET Antarctic Program are financially supported by the Ministry of Science of Spain.
This paper was edited by Andrea Montani and reviewed by two anonymous referees.
Bengtsson, L., Andrae, U., Aspelien, T., Batrak, Y., Calvo, J., De Rooy, W., Gleeson, E., Hansen-Sass, B., Homleid, M., Hortal, M., Ivarsson, K.-I., Lenderink, G., Niemelä, S., Nielsen, K. P., Onvlee, J., Rontu, L., Samuelsson, P., Santos Muñoz, D., Subias, A., Tijm, S., Toll, V., Yang, X., and Køltzow, M. Ø.: The HARMONIE–AROME Model Configuration in the ALADIN–HIRLAM NWP System, Mon. Weather Rev., 145, 1919–1935, https://doi.org/10.1175/MWR-D-16-0417.1, 2017.
Bromwich, D. H., Monaghan, A. J., Manning, K. W., and Powers, J. G.: Real-time forecasting for the Antarctic: An evaluation of the Antarctic Mesoscale Prediction System (AMPS), Mon. Weather Rev., 133, 579–603, https://doi.org/10.1175/MWR-2881.1, 2005.
Bromwich, D. H., Otieno, F. O., Hines, K. M., Manning, K. W., and Shilo, E.: Comprehensive evaluation of polar weather research and forecasting model performance in the antarctic, J. Geophys. Res. Atmos., 118, 274–292, https://doi.org/10.1029/2012JD018139, 2013.
Bromwich, D. H., Werner, K., Casati, B., Powers, J. G., Gorodetskaya, I. V., Massonnet, F., Vitale, V., Heinrich, V. J., Liggett, D., Arndt, S., Barja, B., Bazile, E., Carpentier, S., Carrasco, J. F., Choi, T., Choi, Y., Colwell, S. R., Cordero, R. R., Gervasi, M., Haiden, T., Hirasawa, N., Inoue, J., Jung, T., Kalesse, H., Kim, S.-J., Lazzara, M. A., Manning, K. W., Norris, K., Park, S.-J., Reid, P., Rigor, I., Rowe, P. M., Schmithüsen, H., Seifert, P., Sun, Q., Uttal, T., Zannoni, M., and Zou, X.: The Year of Polar Prediction in the Southern Hemisphere (YOPP-SH), Bull. Am. Meteorol. Soc., preprint, 1–56, https://doi.org/10.1175/bams-d-19-0255.1, 2020.
Dawson, J., Hoke, W., Lamers, M., Liggett, D., Ljubicic, G., Mills, B., Stewart, E., and Thoman, R.: Navigating Weather, Water, Ice and Climate Information for Safe Polar Mobilities, WMO, WWRP/PPP No. 5, 84 pp., 2017.
Ebert, E., Wilson, L., Weigel, A., Mittermaier, M., Nurmi, P., Gill, P., Göber, M., Joslyn, S., Brown, B., Fowler, T., and Watkins, A.: Progress and challenges in forecast verification, Meteorol. Appl., 20, 130–139, https://doi.org/10.1002/met.1392, 2013.
Frogner, I. L., Andrae, U. L. F., Bojarova, J., Callado, A., Escribà, P. A. U., Feddersen, H., Hally, A., Kauhanen, J., Randriamampianina, R., Singleton, A., Smet, G., Van der Veen, S., and Vignes, O. L. E.: HarmonEPS–The HARMONIE ensemble prediction system, Weather Forecast., 34, 1909–1937, https://doi.org/10.1175/WAF-D-19-0030.1, 2019.
Gonzalez, S., Vasallo, F., Recio-Blitz, C., Guijarro, J. A., and Riesco, J.: Atmospheric patterns over the Antarctic Peninsula, J. Climate, 31, 3597–3608, https://doi.org/10.1175/JCLI-D-17-0598.1, 2018.
Hamill, T. M.: Interpretation of rank histograms for verifying ensemble forecasts, Mon. Weather Rev., 129, 550–560, https://doi.org/10.1175/1520-0493(2001)129<0550:IORHFV>2.0.CO;2, 2001.
Hersbach, H.: Decomposition of the continuous ranked probability score for ensemble prediction systems, Weather Forecast., 15, 559–570, https://doi.org/10.1175/1520-0434(2000)015<0559:DOTCRP> 2.0.CO;2, 2000.
Hosking, J. S., Orr, A., Marshall, G. J., Turner, J., and Phillips, T.: The influence of the amundsen-bellingshausen seas low on the climate of West Antarctica and its representation in coupled climate model simulations, J. Climate, 26, 6633–6648, https://doi.org/10.1175/JCLI-D-12-00813.1, 2013.
Janjic, Z. and Gall, R.: Scientific Documentation of the NCEP Nonhydrostatic Multiscale Model on the B grid (NMMB). Part 1 Dynamics, NCAR Tech. Note, NCAR/TN-489+STR, 1–80, https://doi.org/10.5065/D6WH2MZX, 2012.
Jung, T., Gordon, N. D., Bauer, P., Bromwich, D. H., Chevallier, M., Day, J. J., Dawson, J., Doblas-Reyes, F., Fairall, C., Goessling, helge F., Holland, M., Inoue, J., Iversen, T., Klebe, St., Lemke, P., Losch, M., Makshtas, A., Mills, B., Nurmi, P., Perovich, D., Reid, P., Renfrew, I. A., Smith, G., Svensson, G., Tolstykh, M., and Yang, Q.: Advancing polar prediction capabilities on daily to seasonal time scales, B. Am. Meteorol. Soc., 97, 1631–1647, https://doi.org/10.1175/BAMS-D-14-00246.1, 2016.
Monaghan, A. J., Bromwich, D. H., Wei, H. L., Cayette, A. M., Powers, J. G., Kuo, Y. H., and Lazzara, M. A.: Performance of weather forecast models in the rescue of Dr. Ronald Shemenski from the South Pole in April 2001, Weather Forecast., 18, 142–160, https://doi.org/10.1175/1520-0434(2003)018<0142:POWFMI>2.0.CO;2, 2003.
Osmanoglu, B., Navarro, F. J., Hock, R., Braun, M., and Corcuera, M. I.: Surface velocity and mass balance of Livingston Island ice cap, Antarctica, The Cryosphere, 8, 1807–1823, https://doi.org/10.5194/tc-8-1807-2014, 2014.
Powers, J. G., Monaghan, A. J., Cayette, A. M., Bromwich, D. H., Kuo, Y. H., and Manning, K. W.: Real-time mesoscale modeling over Antarctica: The Antarctic mesoscale prediction system, B. Am. Meteorol. Soc., 84, 1533–1546, https://doi.org/10.1175/BAMS-84-11-1533, 2003.
Powers, J. G., Manning, K. W., Bromwich, D. H., Cassano, J. J., and Cayette, A. M.: A decade of antarctic science support through AMPS, B. Am. Meteorol. Soc., 93, 1699–1712, https://doi.org/10.1175/BAMS-D-11-00186.1, 2012.
Quintero Plaza, D. and García-Moya Zapata, J. A.: Statistical Postprocessing of Different Variables for Airports in Spain Using Machine Learning, Adv. Meteorol., 2019, 3181037, https://doi.org/10.1155/2019/3181037, 2019.
Recio-Blitz, C., Navarro, F. J., Otero, J., Lapazaran, J., and Gonzalez, S.: Effects of recent cooling in the antarctic peninsula on snow density and surface mass balance, Pol. Polar Res., 39, 457–480, https://doi.org/10.24425/118756, 2018.
Santos, C.: Física del caos en la predicción meteorológica, edited by: Santos, C., AEMET, Madrid, 2018.
Skamarock, W. C., Klemp, J. B., Dudhi, J., Gill, D. O., Barker, D. M., Duda, M. G., Huang, X.-Y., Wang, W., and Powers, J. G.: A Description of the Advanced Research WRF Version 3, NCAR Tech. Note NCAR/TN-475+STR, 113 pp., https://doi.org/10.5065/D68S4MVH, 2008.
Termonia, P., Fischer, C., Bazile, E., Bouyssel, F., Brožková, R., Bénard, P., Bochenek, B., Degrauwe, D., Derková, M., El Khatib, R., Hamdi, R., Mašek, J., Pottier, P., Pristov, N., Seity, Y., Smolíková, P., Španiel, O., Tudor, M., Wang, Y., Wittmann, C., and Joly, A.: The ALADIN System and its canonical model configurations AROME CY41T1 and ALARO CY40T1, Geosci. Model Dev., 11, 257–281, https://doi.org/10.5194/gmd-11-257-2018, 2018.