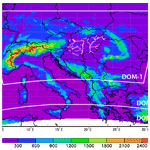
Sensitivity study of the REMO regional climate model to domain size
Réka Suga
Otília A. Megyeri-Korotaj
Gabriella Allaga-Zsebeházi
In the framework of the KlimAdat national project, the Hungarian Meteorological Service (OMSZ) is aiming to perform 10 km horizontal resolution simulations with the 2015 version of the REMO regional climate model over Central and Eastern Europe. The long-term simulations were preceded by a 10-year long sensitivity study on domain size, which is summarised in this paper. We selected three different domains embedded in each other, which contain the whole area of the Danube and Tisza river catchments. Lateral boundary conditions were obtained from the 50 km resolution REMO driven by the MPI-ESM-LR global climate model. Simulations were performed for the period of 1970–1980 including 1-year spin-up. Monthly and seasonal means of daily 2 m temperature, precipitation sum and several precipitation indices were evaluated. Reference datasets were E-OBS 19.0 and CarpatClim-HU. We can conclude, that the selection of domain size has a larger impact on the simulation of precipitation, and in the case of the seasonal mean of the precipitation indices, the differences amongst the results obtained on each model domain exceed 10 %. In general, the smallest biases occurred on the largest domain, therefore further long-term simulations are being produced on this domain.
- Article
(15226 KB) - Full-text XML
- BibTeX
- EndNote
The REMO 5.0 (REgional MOdel, Jacob and Podzun, 1997; Jacob et al., 2007) regional climate model (RCM) was adapted at the Hungarian Meteorological Service in 2004 besides the ALADIN-Climate model. The two adapted RCMs enabled to obtain reliable, quantitative, detailed information about climate change and its uncertainties in Hungary. As a first step, short, few year-long experiments were implemented with REMO to define the fundamental settings for operational work. Then, in the framework of EU FP6 project CLAVIER, 25 km resolution simulations were implemented for the 21st century over Central and Eastern Europe (Szépszó and Horányi, 2008). The integration domain and the resolution were specified as a trade-off between the computational capacity and the needs of the impact studies (e.g., in the field of hydrology, agriculture).
Within the framework of KlimAdat national project, funded by the EU Cohesion Fund, the Hungarian Meteorological Service has been extending its regional projections adapting recent model versions of REMO and ALADIN-Climate and applying finer resolution and new anthropogenic scenarios to better fulfil the user needs in Hungary. Although several simulations are available on similar resolution (12.5 km) in the Euro-CORDEX framework, the publicly accessible data is mainly confined to surface and pressure level variables on hourly frequency at most. However, certain models (e.g., land surface models) require model level data on sub-hourly frequency as forcings that cannot be obtained even upon request, since such data is usually not stored at the modelling centres. Our final goal is to develop an own set of simulations, that is (1) suited for the Carpathian Basin, to serve impact modellers and decision makers needs and (2) that is appropriate to further downscale over cities or lakes with a land surface model. In KlimAdat, the Hungarian Meteorological Service has already set up 10 km resolution simulations with ALADIN-Climate (Bán et al., 2021) and the results were used as an input for SURFEX (Zsebeházi and Szépszó, 2020). The same methodology is followed with the recent version of REMO, REMO2015 (Jacob et al., 2012; Pietikäinen et al., 2018; Top et al., 2020) and as first step, we have made a sensitivity study to determine the best location and size of the integration domain of REMO.
The choice of model setup (e.g., domain size, and its location, horizontal resolution) can cause uncertainties in the results. A brief overview is given about studies related to domain selection problem:
-
It is appropriate to choose the boundaries of the domain at least a few grid points further from the edges of the target area (Szépszó, 2014; Szépszó et al., 2015; Seth and Giorgi, 1998) and avoid the cross of mountainous areas. The lateral boundary conditions (provided by either global climate models, by an RCM applied on a bigger domain containing the target area or by reanalysis) are smoothed together with the regional model fields in a few grid points at the boundaries of the model domain using a relaxation technique (e.g., Davies, 1976). As a result, in the relaxation zone non-realistic features, noises can appear – which in case of a smaller domain – might spread into the target area.
-
As indicated above, boundary conditions exert outer constraints on regional model through relaxation zone only. In this part, the large-scale effects of global models interact with the local small-scale processes (e.g. orography, surface turbulent fluxes, convection). Using a smaller domain, the constraints caused by boundary conditions are bigger. Small-scale processes require a relatively big domain to develop properly (Jones et al., 1995).
-
As Larsen et al. (2013) has proved through a sensitivity study with HIRHAM regional climate model, that – taken into account computational capacity limits – increasing the size of the model domain is more important in the case of precipitation, than increasing its resolution.
Nevertheless, selecting the domain size and its position require careful investigation based on the modelling task and key points described above. In order to eliminate this uncertainty, on international level the WCRP-CORDEX initiative has been established, which European branch (the Euro-CORDEX; Jacob et al., 2014, 2020) gathers the European regional climate modelling activities under a common umbrella to perform the simulations over the same domain and on the same resolution.
Due to the uncertainties indicated above, we made some sensitivity tests with REMO2015 preceding the long-term simulations to define the best setting of the model domain. This investigation is based on the previous sensitivity study of Szépszó et al. (2015), where it has been proved that on a bigger domain noises in the target area coming from the lateral boundary conditions' handling are less relevant than the error coming from the driving model compared to choosing a smaller domain. Besides the abovementioned aspects of numerical modelling theory, the location and extent of model domain were investigated from the point of applicability of the results in various impact studies as well (e.g., covering river catchments for hydrological studies).
In the next section (Sect. 2) we will introduce the methodology, including the model and experimental design (Sect. 2.1), the reference datasets and the evaluation methods (Sect. 2.2). Section 3 provides a detailed analysis of the results for temperature (Sect. 3.1) and precipitation (Sect. 3.2). Finally, a summary and outlook are given in Sect. 4.
2.1 Model and experimental design
REMO is a hydrostatic, gridpoint regional climate model that is developed at the Climate Service Center Germany (GERICS). The dynamics of the model is originated from the German Weather Service's (DWD's) former weather forecasting model, the Europa-Model (Majewski, 1991), while the physical parameterisation was derived from the ECHAM4 (Roeckner et al., 1996) atmospheric circulation model.
At the Hungarian Meteorological Service we are currently using the 2015 version of REMO. The prognostic variables of the model are horizontal wind components, surface air pressure, temperature, specific humidity, cloud ice and cloud water content. The model solves the equations in a rotated spherical coordinate system. In the horizontal direction, it uses the spherical Arakawa-C grid (Arakawa and Lamb, 1977), which calculates the prognostic variables in the middle of the grid cell, with the exception of the wind components, that are calculated at its boundaries. In the vertical direction, it uses a hybrid coordinate system. The temporal discretisation is done by the leapfrog scheme with semi-implicit correction and Asselin-filter (Asselin, 1972). The boundary conditions and the REMO integration results are smoothed out in a relaxation zone (Davies, 1976) that is composed of 8 grid points. The heterogeneity of surface cover is taken into account based on the tiling method, with three tiles: land, water and ice (Semmler et al., 2004).
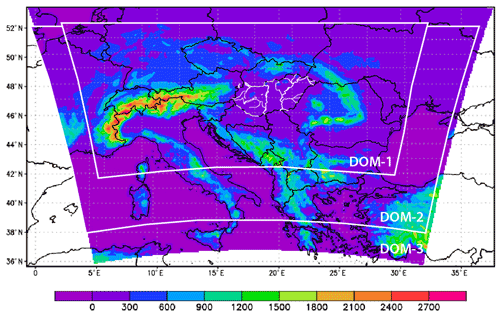
Figure 1Area and topography of the three selected model domains (altitude above sea level, m). For Hungary the rivers and lakes are indicated with white colour.
For the sensitivity study, REMO was run on 0.09∘ (approximately 10 km) horizontal resolution, with 27 vertical levels and 30 s time step. The following considerations were taken into account when designing the sensitivity test:
-
The length of the experiment should be suitable for evaluating the results on climate timescale, where the role of natural variability is smaller,
-
The size and location of the domains should be suitable for a wide range of impact studies,
-
The number of simulations is limited by the computing capacity.
Therefore, we selected three domains, based on our previous studies with REMO and the results of former ALADIN sensitivity studies (Szépszó et al., 2015; Fig. 1, Table 1). The largest domain (DOM-3) is identical to the domain of REMO5.0 previously used in the CLAVIER project, while the smallest (DOM-1) and medium (DOM-2) domains are similar to those used in the ALADIN sensitivity test. All three areas cover Central and Eastern Europe, including the catchment areas of the Danube and Tisza rivers. The DOM-3 domain includes DOM-2 and DOM-2 includes DOM-1. DOM-3 mainly extends south from DOM-2, while DOM-2 extends farther east and south from DOM-1. The decade of 1971–1980 was chosen as the test period with a one-year spin-up (1970).
The boundary conditions for the model simulations were derived from the 50 km resolution REMO model simulation (hereinafter REMO_0.44; Teichmann et al., 2013) performed by the Climate Service Center in the framework of the Euro-CORDEX initiative. REMO_0.44 was driven by the MPI-ESM-LR r1i1p1 global model in a region covering Europe and North Africa (EUR-44). Our aim was to find the most appropriate model domain set-up from the aspect of future climate projections. For the future, we will drive REMO with REMO_0.44 as well, thus the conclusions drawn from the sensitivity study provide solid information about the settings for long-term experiments.
2.2 Reference datasets and evaluation methods
Two reference databases were used for the sensitivity studies: E-OBS version 19.0 (Cornes et al., 2018; published in March 2019) and CarpatClim-HU (Bihari et al., 2017) databases.
The E-OBS database contains daily surface observation data for Europe on 10 km horizontal resolution. It is mostly prepared by interpolating publicly available station datasets for several meteorological variables. Due to its European coverage, it is mostly used for large-scale analysis of model results. Its values for Hungary (especially precipitation data) often show significant differences from the domestic database (Kotlarski et al., 2017; Illy et al., 2015), which can be explained by fewer station data sets, lack of homogenisation and different interpolation methods. CarpatClim-HU database contains daily surface observation data generated with homogenisation and interpolation methods specially developed for meteorological purposes (Szentimrey, 2008; Szentimrey and Bihari, 2007) on a 10 km resolution grid covering Hungary. It has a key role in the validation studies for Hungary, as for the development of CarpatClim-HU as many station datasets as possible were used, thus it provides the most accurate information about past climate.
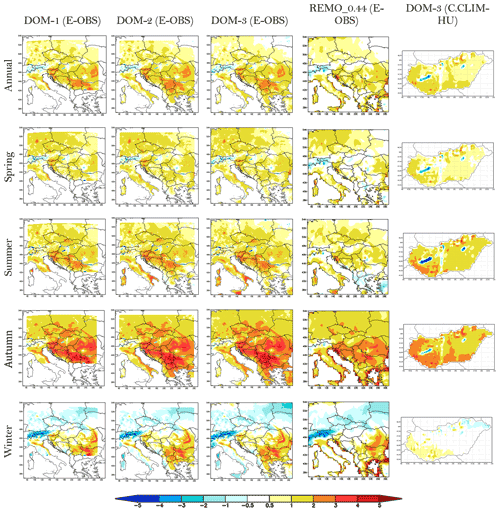
Figure 2The annual and seasonal temperature bias (∘C) of the REMO model simulations on DOM-1, DOM-2 and DOM-3, and of REMO_0.44 compared to the E-OBS observational dataset (first four columns) and of the DOM-3 experiment compared to CarpatClim-HU (last column). Period: 1971–1980.
In the analysis, annual, seasonal and monthly mean of daily mean temperature and precipitation sum were calculated for the model domain and for Hungary. Although considering more variables would have an additional added value on this study, the lack of appropriate and high-quality reference data makes it difficult to use (e.g., daily mean windspeed in CarpatClim-HU was computed from 3 measurements per day). In addition, temperature and precipitation statistics are the most often required data by the users. Since precipitation is a highly variable parameter, describing it with models is a more complex task. Therefore, daily precipitation statistics were also evaluated with several precipitation indices derived from the ETCCDI (Expert Team on Climate Change Detection and Indices (http://etccdi.pacificclimate.org/, last access: 20 September 2021)), namely wet days (RR ≥ 1 mm; RR1), heavy precipitation days (RR ≥ 10 mm; RR10), very heavy precipitation days (RR ≥ 20 mm; RR20), and the maximum number of consecutive dry days (RR < 1 mm; CDD). The spatial distribution of differences between the model results and the observations is shown on maps for the whole domains and Hungary. In addition, spatial average of annual, seasonal and monthly means for Hungary is presented in tables and graphs. The results of the experiments performed in three different domains were also directly compared with each other in order to explore the differences in more detail.
3.1 Temperature
First of all, we analysed the 2 m temperature parameter as follows. In general, from spring to autumn there are an average 0.5–2 ∘C overestimation over the whole domains, in comparison with E-OBS dataset. In autumn, we can see the largest overestimation of the REMO model (Fig. 2): 2–4 ∘C positive bias spans from Czech Republic through Central-Europe to Balkan countries. In summer and spring, this difference is slightly weaker. In winter, north from the Carpathian Basin 1–2 ∘C and in the Alps 3–4 ∘C underestimation is visible.
From spring to autumn, lakes and rivers (e.g. Balaton, Danube) seem much colder than their surrounding area in the REMO model (Fig. 2). This pattern can be partly explained by the inconsistency of observations and REMO. In the observation datasets (E-OBS and CarpatClim-HU) water temperatures are interpolated from the nearest terrestrial data. However, the REMO model takes into account lakes and rivers with a simple method where the surface temperature of the nearest sea point is given for all inland water and sea fractions of land use types (Pietikäinen et al., 2018). Nevertheless, the model is able to describe the cooling effect of lakes and rivers on near-surface temperature. It is not known how precise its performance over the Carpathian Basin due to the caveats of the observation dataset, but according to the abovementioned study, this method is sufficient for regions with low fractional area of lakes.
Table 2Annual and seasonal temperature bias (∘C) between the three model simulations and of REMO_0.44 compared to the E-OBS (first four columns), and between the 10 km model simulations and CarpatClim-HU data (last three columns). Temperature data above lakes and rivers is not taken into account in the spatial averages of REMO.
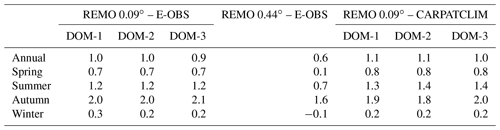
Looking at the results compared to CarpatClim-HU dataset over Hungary, the smallest bias appears in spring and winter (0.8 and 0.2 ∘C respectively), while the biggest bias was detected in autumn (1.8–2.0 ∘C; Table 2). In the last panel of Fig. 2, we can see the seasonal bias pattern in detail, thus the southwestern part of the country appears with the most dominant positive difference (1–3 ∘C, depending on the season). Besides, the areas with higher elevation suffer from considerable positive bias as well. In addition, since E-OBS is slightly warmer than CarpatClim-HU, the positive bias with the latter observation dataset is bigger than with the European dataset (Table 2).
Comparing the downscaled model results to the 50 km REMO simulation, we can conclude that the latter gave better performance over Hungary in spring and summer than the finer resolution simulations (Table 2 and Fig. 2), because the 10 km REMO simulations add extra warming to their driving fields. Investigating the differences between the domains (Fig. 3), we can see that biases slightly differ from each other (with 0.1 ∘C), therefore, based on temperature data, we cannot conclude which domain is significantly better to use for further investigations.
3.2 Precipitation
REMO overestimates the seasonal mean precipitation sum basically in all seasons, but mostly in spring and winter (partially with more than 50 %) compared to E-OBS (Fig. 4). However, above the Carpathians, the Apennines, and the Dinaric Alps and in the southern European plains, the modelled precipitation is too small in summer and autumn. If we compare the results of REMO with CarpatClim-HU (Fig. 4), we have to make some adjustments to our previous findings, as CarpatClim-HU is on average 5–10 mm wetter than E-OBS in each month (not shown). As a result, the average error over Hungary is more than 25 % lower in winter, 15 %–20 % lower in spring and autumn, and in spring in the south-western parts of the country the sign of the error has reversed (Table 3).
Table 3Annual and seasonal relative precipitation bias (%) between the three model simulations and REMO_0.44 compared to the E-OBS (first four columns) and between the 10 km model simulations and CarpatClim-HU data (last three columns). Precipitation data above lakes and rivers is not taken into account in the spatial averages of REMO. In case of differences in mean bias between DOM-1 or DOM-2 and DOM-3 reach 5 %, it is indicated with bold.
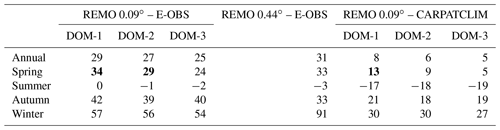
While summer proved to be the season modelled with the smallest bias compared to E-OBS over Hungary, the season is too dry (−17 % to −19 %) compared to CarpatClim-HU. Over the Lake Balaton and the Danube, presumably due to the spurious behaviour of parameterisation over the water surfaces, the model's precipitation underestimation exceeds 50 %.
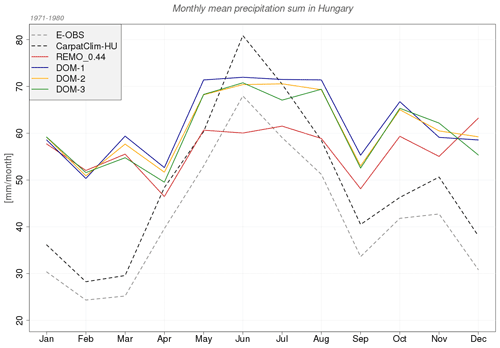
Figure 5The average monthly precipitation amounts (mm/month) for Hungary in the period of 1971–1980 based on the results of the various REMO model simulations and the data of the CarpatClim-HU and E-OBS reference databases.
Examining the average monthly precipitation (Fig. 5), it can be said that the model simulations underestimate the seasonal variability of precipitation in Hungary, mainly due to the significant winter precipitation surplus. From May to August, the monthly amounts are almost the same, and June does not rise as the wettest month contrary to observation. Due to the differences between the two reference databases, the simulated sum in July is too much compared to E-OBS and too small compared to CarpatClim-HU. Although, from May to August the 10 km resolution simulations produce much more precipitation than REMO_0.44, resulting in a better description of seasonal variability compared to the LBC.
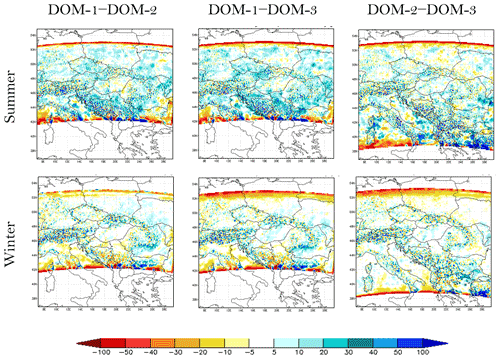
Figure 6Relative difference (%) of the amount of summer and winter precipitation between the results of the experiments performed on different domains. Period: 1971–1980.
Comparing the simulations with each other (Fig. 6, summer and winter results are shown), only minor differences can be seen for Hungary. Mostly south from the Carpathian Basin REMO resulted in 10 %–20 % (in some places 20 %–30 %) more precipitation in the smaller integration domain, especially in spring and summer compared to the results of the larger domain, whereas in autumn and winter there were only small differences between the three simulations. The largest difference is observed between the DOM-1 and DOM-3 domains (seasonally between |2 %–8 %| for Hungary, the largest in spring; Table 3), while the smallest (−1 %–4 %) occurs between DOM-2 and DOM-3 (note, that in this case there are smaller differences between the domain sizes too). Overall, with the exception of summer, the experiment in the DOM-3 domain performed the best, but in summer, due to the more precipitation in the smaller domain (and due to the general underestimation compared to CarpatClim-HU), we obtained the smallest errors by choosing DOM-1 (Fig. 6).
Table 4The relative bias (%) of the annual and seasonal averages of the different precipitation indices between the results of the REMO model simulations and the CarpatClim-HU data in the period 1971–1980 for Hungary. The model experiments describing the given indices in a given season with the smallest error were marked (*: DOM-1, **: DOM-2, ***: DOM-3). In the last column of the table, we summarised how many seasons the given domain proved to be the best for a given index. In addition, if the deviation between the biases for all experiments exceeds 10 % in relative terms for a given index in a given season, it is indicated in bold. The given indices are the follows: CDD (maximum no. of consecutive dry days, RR < 1 mm), RR1 (no. of wet days, RR ≥ 1 mm), RR10 (heavy precipitation days, RR ≥ 10 mm) and RR20 (very heavy precipitation days, RR ≥ 20 mm).
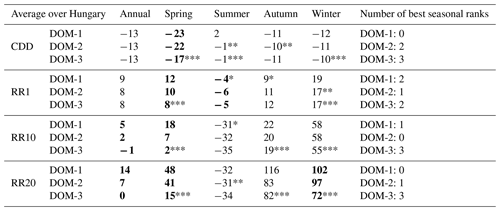
In the following, the evaluation of precipitation indices is shown with respect to the CarpatClim-HU reference dataset. Looking at the daily precipitation amounts, it also supports our previous findings of the overestimation of precipitation in the REMO model. The modelled precipitation indices referring to the daily precipitation distribution (RR1, RR10, RR20) are more frequent in all seasons except summer and less frequent in summer compared to CarpatClim-HU data (Table 4). In general, the largest bias can be observed in winter, and in the case of RR20 in autumn as well. The seasonal mean bias of RR1 does not exceed 20 % for each domain; however, for RR20 the bias mostly exceeds 40 % and in winter and autumn it is larger than 70 %. Examining the annual number of the RR10 index, we can see that the model bias does not exceed 5 % considering the spatial means, nevertheless in the eastern part of the country model values are 10 %–30 % higher than the observed averages, while in the western part of the country they are underestimated by about the same amount (not shown). In summer, 10 and 20 mm daily precipitation events are 31 %–35 % less frequent in the REMO than observation considering the different domains.
The average annual length of the longest dry periods (CDD) in the model results is 13 % longer than the observation (Table 4), on the other hand, in the eastern and western areas of Hungary 10 %–30 % shorter periods are simulated (not shown). The largest bias [−17 % to −23 %] occurs in spring, while in summer the near-perfect spatial means hide the controversies of different signs of errors over the country.
Experiments performed in different domains show greater differences in the daily precipitation amounts (sometimes even exceeding 10 % on average); however, considering different indices and seasons, different experiments prove to be the best. Based on the examination of the average errors, we can conclude that most often the result from the largest (DOM-3) domain have the smallest errors. This conclusion largely underpinned our decision to conduct further long-term experiments in the DOM-3 domain.
Our findings are in good agreement with several other researches focussing on determining the influence of domain size on regional climate models' performance. E.g., Leduc and Laprise (2008) and Laprise et al. (2012) showed through a perfect model approach called Big Brother Experiment, that a relatively large domain (larger than 100×100 gridpoint) is needed in order to develop the small scale atmospheric features properly. However, Seth and Giorgi (1998) performed 3-month simulations in summer with the RegCM regional climate model over the upper Mississippi basin using different size domains and obtained better precipitation results with the smaller domain. Vannitsem and Chomé (2005) – applying the Eta model for 20 d simulations choosing several different size model domains centred around Western Europe – also found the that more realistic summer precipitation may be simulated over a smaller domain.
At the Hungarian Meteorological Service within the framework of the KlimAdat project, we have implemented a sensitivity study on domain selection with REMO2015 regional climate model. The goal of the experiment was to find the optimal domain size and location for further projection experiments. Therefore, the boundary conditions for the model simulations were derived from the REMO_0.44 model simulation, which was driven by the MPI-ESM-LR global model, because future climate change projections will be performed with the same boundary conditions.
Three different domains were selected which cover Central and South-Eastern Europe including the whole Danube and Tisza catchment area. We evaluated 2 m temperature and total precipitation against CarpatClim-HU and E-OBS observation datasets. For better understanding the simulation of the daily precipitation distribution, we calculated and compared different precipitation indices as well.
As a result, we can conclude that REMO is warmer than observation in each season except winter, especially in autumn, independently from the applied integration domain. The biases are slightly bigger to CarpatClim-HU than to E-OBS and only minor differences were found between the results achieved on the individual domains.
Regarding the precipitation, REMO is generally too moist over Hungary, mostly in winter. However, in summer, thanks to CarpatClim-HU is wetter than E-OBS we obtained the smallest bias according to E-OBS, while large (20 %–40 %) underestimation compared to CarpatClim-HU. For daily precipitation indices biases were especially high (usually more than 10 %) and different indices yielded the smallest bias in different seasons. The selection of domain size has a large impact on the simulation of precipitation, and the differences often exceed 10 % for seasonal mean precipitation indices. In general, the smallest differences were obtained on the largest (DOM-3) domain, therefore further long term simulations are achieved on this.
The conclusions of the present study coincide with Szépszó et al. (2015), who executed similar sensitivity study for the ALADIN-Climate regional climate model. The biases of the ALADIN-Climate model on a 10-year period are comparable with REMO (e.g., seasonal temperature bias is between −2 and 3 ∘C, precipitation bias is between −20 % and 30 %). Vautard et al. (2020) evaluated 55 Euro-CORDEX GCM-RCM combinations including MPI driven REMO for different regions of Europe for 1981–2010. They also found that REMO overestimates the summer temperature over Eastern Europe and the Mediterranean region with less than 1 ∘C; however, the winter overestimation is larger than 1 ∘C over the Mediterranean. Too much precipitation is simulated throughout Europe, except Eastern Europe in summer, when slight underestimation occurred. The sensitivity study will be followed by the model validation, when two 10 km resolution simulations are performed using different lateral boundary conditions: ERA-Interim reanalysis data (for 1980–2005; evaluation run) and the MPI-ESM-LR driven REMO on the EUR44 domain (for 1950–2005; historical run). This validation study will reveal the regional model biases itself (using the reanalysis as LBC) and the longer historical simulation can underpin or modify the statements of the evaluation results obtained in this sensitivity study.
The output files of REMO simulations and the CarpatClim-HU observational database have been stored by the authors and they are available upon request. E-OBS 19.0 can be downloaded from the C3S SurfObs webpage (C3S Climate Data Store, 2019) (https://doi.org/10.24381/cds.151d3ec6).
The authors planned and wrote the manuscript. RS and GAZ designed the experiments, and RS carried them out. RS and OAMK performed the analysis.
The authors declare that they have no conflict of interest.
Publisher's note: Copernicus Publications remains neutral with regard to jurisdictional claims in published maps and institutional affiliations.
This article is part of the special issue “Applied Meteorology and Climatology Proceedings 2020: contributions in the pandemic year”.
The authors would like to thank to Lars Buntemeyer (GERICS) for his support in the installation of the REMO and the first steps of the simulation. We would also like to thank him for providing the lateral boundary condition files for REMO. Last, but not least, we would like to thank the two anonymous reviewers who improved the quality of the article with their comments.
This research has been supported by the KlimAdat project in the framework of the Environmental and Energy Efficiency Operative Program (KEHOP) funded by the Hungarian Government and the European Union Cohesion Fund. (grant no. KEHOP-1.1.0-15-2015-00001).
This paper was edited by Emily Gleeson and reviewed by four anonymous referees.
Arakawa, A. and Lamb, V.: Computational design and the basic dynamical processes of the UCLA general circulation Model, Methods in Computational Physics, 17, 173–265, 1977.
Asselin, R.: Frequency filter for time integrations, Mon. Weather Rev., 100, 487–490, 1972.
Bán, B., Király, A., Szépszó, G., Somot S., and Zsebeházi, G.: ALADIN-Climate at the Hungarian Meteorological Service: from the beginnings to present days, Időjárás, in review, 2021.
Bihari, Z., Lakatos, M., and Szentimrey, T.: Gridded observational datasets based on surface observations at the Hungarian Meteorological Service, Légkör, 62, 148–151, 2017 (in Hungarian).
C3S Climate Data Store: E-OBS daily gridded meteorological data for Europe from 1950 to present derived from in-situ observations, C3S Climate Data Store [data set], https://doi.org/10.24381/cds.151d3ec6, 2019.
Cornes, R., van der Schrier, G., van den Besselaar, E. J. M., and Jones, P. D.: An Ensemble Version of the E-OBS Temperature and Precipitation Datasets, J. Geophys. Res.-Atmos., 123, 9391–9409, https://doi.org/10.1029/2017JD028200, 2018.
Davies, H. C.: A lateral boundary formulation for multi-level prediction models, Q. J. Roy. Meteor. Soc., 102, 405–418, https://doi.org/10.1002/qj.49710243210, 1976.
Illy, T., Sábitz, J., and Szépszó, G.: Validation of the ALADIN-Climate model experiments, RCMTéR (EEA-C13-10) project report, Hungarian Meteorological Service, Budapest, 19 pp., 2015 (in Hungarian).
Jacob, D. and Podzun, R.: Sensitivity studies with the regional climate model REMO, Meteor. Atmos. Phys., 63, 119–129, https://doi.org/10.1007/BF01025368, 1997.
Jacob, D., Bärring, L., Christensen, O. B., Christensen, J. H., Castro, M. D., Déqué, M., Giorgi, F., Hagemann, S., Hirschi, M., Jones, R., Kjellström, E., Lenderink, G., Rockel, B., Sánchez, E., Schär, C., Seneviratne, S. I., Somo, S., Ulden, A. V., Hurk, B. V. D.: An inter-comparison of regional climate models for Europe: model performance in present-day climate, Clim. Change, 81, 31–52, https://doi.org/10.1007/s10584-006-9213-4, 2007.
Jacob, D., Elizalde, A., Haensler, A., Hagemann, S., Kumar, P., Podzun, R., Rechid, D., Remedio, A.R., Saeed, F., Sieck, K., Teichmann, C., and Wilhelm, C.: Assessing the Transferability of the Regional Climate Model REMO to Different COordinated Regional Climate Downscaling EXperiment (CORDEX) Regions, Atmosphere, 3, 181–199, https://doi.org/10.3390/atmos3010181, 2012.
Jacob, D., Petersen, J., Eggert, B., Alias, A., Christensen, O. B., Bouwer, L. M., Braun, A., Colette, A., Déqué, M., Georgievski, G., Georgopoulou, E., Gobiet, A., Menut, L., Nikulin, G., Haensler, A., Hempelmann, N., Jones, C., Keuler, K., Kovats, S., Kröner, N., Kotlarski, S., Kriegsmann, A., Martin, E., Meijgaard, E. V., Moseley, C., Pfeifer, S., Preuschmann, S., Radermacher, C., Radtke, K., Rechid, D., Rounsevell, M., Samuelsson, P., Somot, S., Soussanna, J.-F., Teichmann, C., Valentini, R., Vautard, R., Weber, B., and Yiou, P.: EURO-CORDEX: new high-resolution climate change projections for European impact research, Reg. Environ. Change, 14, 563–578, https://doi.org/10.1007/s10113-013-0499-2, 2014.
Jacob, D., Teichmann, C., Sobolowski, S., Katragkou, E., Anders, I., Belda, M., Benestad, R., Boberg, F., Buonomo, E., Cardoso, R. M., Casanueva, A., Christensen, O. B., Christensen, J. H., Coppola, E., Cruz, L. D., Davin, E. L., Dobler, A., Domínguez, M., Fealy, R., Fernandez, J., Gaertner, M. A., García-Díez, M., Giorgi, F., Gobiet, A., Goergen, K., Gómez-Navarro, J. J., Alemán, J. J. G., Gutiérrez, C., Gutiérrez, J. M., Güttler, I., Haensler, A., Halenka, T., Jerez, S., Jiménez-Guerrero, P., Jones, R. G., Keuler, K., Kjellström, E., Knist, S., Kotlarski, S., Maraun, D., Meijgaard, E. V., Mercogliano, P., Montávez, J. P., Navarra, A., Nikulin, G., Noblet-Ducoudré, N. D., Panitz, H.-J., Pfeifer, S., Piazza, M., Pichelli, E., Pietikäinen, J.-P., Prein, A. F., Preuschmann, S., Rechild, D., Rockel, B., Romera, R., Sánchez, E., Sieck, K., Soares, P. M. M., Somot, S., Srnec, L., Sørland, S. L., Termonia, P., Truhetz, H., Vautard, R., Wrrach-Sagi, K., and Wulfmeyer, V.: Regional climate downscaling over Europe: perspectives from the EURO-CORDEX community, Reg. Environ. Change, 20, 51, https://doi.org/10.1007/s10113-020-01606-9, 2020.
Jones, R. G., Murphy, J. M., and Noguer, M.,: Simulation of climate change over Europe using a nested regional climate model. I: Assessment of control climate, including sensitivity to location of lateral boundaries, Q. J. Roy. Meteor. Soc., 121, 1413–1449, https://doi.org/10.1002/qj.49712353802, 1995.
Kotlarski, S., Szabó, P., Herrera, S., Räty, O., Keuler, K., Soares, P. M., Cardoso, R. M., Bosshard, T., Pagé, C., Boberg, F., Gutiérrez, J. M., Isotta, F. A., Jaczewski, A., Kreienkamp, F., Liniger, M. A., Lussana, C., and Pianko-Kluczyńska, K.: Observational uncertainty and regional climate model evaluation: A pan-European perspective, Int. J. Climatol., 39, 3730–3749, https://doi.org/10.1002/joc.5249, 2017.
Laprise, R., Kornic, D., Rapaić, M., Šeparović, L., Leduc, M., Nikiema, O., Di Luca, A., Diaconescu, E., Alexandru, A., Lucas-Picher, P., de Elía, R., Caya, D., and Biner, S.: Considerations of Domain Size and Large-Scale Driving for Nested Regional Climate Models: Impact on Internal Variability and Ability at Developing Small-Scale Details, in: Climate Change, edited by: Berger, A., Mesinger, F., and Sijacki, D., Springer, Vienna, 181–199, https://doi.org/10.1007/978-3-7091-0973-1_14, 2012.
Larsen, M. A. D., Thejll, P., Christensen, J. H., Refsgaards, J. C., and Jensen, K.: On the role of domain size and resolution in the simulations with the HIRHAM regional climate model, Clim. Dynam., 40, 2903–2918, 2013.
Leduc, M. and Laprise, R.: Regional climate model sensitivity to domain size, Clim. Dynam., 32, 833–854, https://doi.org/10.1007/s00382-008-0400-z, 2009.
Majewski, D.: The Europa Modell of the Deutscher Wetterdienst, Conference Paper, ECMWF Seminar of Numerical Methods in Atmospheric Models II, ECMWF, Shinfield Park, Reading, 147–191, 1991.
Pietikäinen, J.-P., Markkanen, T., Sieck, K., Jacob, D., Korhonen, J., Räisänen, P., Gao, Y., Ahola, J., Korhonen, H., Laaksonen, A., and Kaurola, J.: The regional climate model REMO (v2015) coupled with the 1-D freshwater lake model FLake (v1): Fenno-Scandinavian climate and lakes, Geosci. Model Dev., 11, 1321–1342, https://doi.org/10.5194/gmd-11-1321-2018, 2018.
Roeckner, E., Arpe, K., Bengtsson, L., Christoph, M., Claussen, M., Dümenil, L., Esch, M., Giorgetta, M., Schlese, U., and Schulzweida, U.: The atmospheric general circulation model ECHAM-4: Model description and simulation of present-day climate, Report No. 18, Max Planck Institute for Meteorology, Hamburg, Germany, 1996.
Semmler, T., Jacob, D., Schlünzen, K. H., and Podzun, R.: Influence of sea ice treatment in a regional climate model on boundary layer values in the Fram Strait region, Mon. Weather Rev., 132, 985–999, 2004.
Seth, A. and Giorgi, F.: The Effects of Domain Choice on Summer Precipitation Simulation and Sensitivity in a Regional Climate Model, J. Climate, 11, 2698–2712, 1998.
Szentimrey, T.: Development of MASH homogenization procedure for daily data, Proceedings of the Fifth Seminar for Homogenization and Quality Control in Climatological Databases, Budapest, Hungary, 2006 WCDMP-No. 71, WMO/TD-NO. 1493, 123–130, 2008.
Szentimrey, T. and Bihari, Z.: Mathematical background of the spatial interpolation methods and the software MISH (Meteorological Interpolation based on Surface Homogenized Data Basis), in: Proceedings of the Conference on Spatial Interpolation in Climatology and Meteorology, edited by: Szalai, S., Bihari, Z., Szentimrey, T., and Lakatos, M., COST Office, Luxemburg, ISBN 92-898-0033-X, 17–28, 2007.
Szépszó, G.: Climate Dynamics investigations for the Carpathian Basin with REMO regional climate model, PhD thesis, Eötvös Loránd University, Department of Meteorology, 162 pp., 2014 (in Hungarian).
Szépszó, G. and Horányi, A.: Transient simulation of the REMO regional climate model and its evaluation over Hungary, Időjárás, 112, 203–231, 2008.
Szépszó, G., Krüzselyi, I., Illy, T., and Sábitz, J.: Sensitivity study of the ALADIN-Climate regional climate model to domain selection, RCMTéR (EEA-C13-10) project report, Hungarian Meteorological Service, Budapest, 19 pp., 2015 (in Hungarian).
Teichmann, C., Eggert, B., Elizalde, A., Haensler, A., Jacob, D., Kumar, P., Moseley, C., Pfeifer, S., Rechid, D., Remedio, A.R., Ries, H., Petersen, J., Preuschmann, S., Raub, T., Saeed, F., Sieck, K., and Weber, T.: How Does a Regional Climate Model Modify the Projected Climate Change Signal of the Driving GCM: A Study over Different CORDEX Regions Using REMO, Atmosphere, 4, 214–236, 2013.
Top, S., Kotova, L., De Cruz, L., Aniskevich, S., Bobylev, L., De Troch, R., Gnatiuk, N., Gobin, A., Hamdi, R., Kriegsmann, A., Remedio, A. R., Sakalli, A., Van De Vyver, H., Van Schaeybroeck, B., Zandersons, V., De Maeyer, P., Termonia, P., and Caluwaerts, S.: Evaluation of regional climate models ALARO-0 and REMO2015 at 0.22∘ resolution over the CORDEX Central Asia domain, Geosci. Model Dev., 14, 1267–1293, https://doi.org/10.5194/gmd-14-1267-2021, 2021.
Vannitsem, S. and Chomé, F.: One-Way Nested Regional Climate Simulations and Domain Size, J. Climate, 18, 229–233, https://doi.org/10.1175/JCLI3252.1, 2005.
Vautard, R., Kadygrov, N., Iles, C., Boberg, F., Buonomo, E., Bülow, K., Coppola, E., Corre, L., van Meijgaard, E., Nogherotto, R., Sandstad, M., Schwingshackl, C., Somot, S., Aalbers, E., Christensen, O. B., Ciarlò, J. M., Demory, M.-E., Giorgi, F., Jacob, D., Jones, R. G., Keuler, K., Kjellström, E., Lenderink, G., Levavasseur, G., Nikulin, G., Sillmann, J., Solidoro, C., Sørland, S. L., Steger, C., Teichmann, C., Warrach-Sagi, K., and Wulfmeyer, V.: Evaluation of the large EURO-CORDEX regional climate model ensemble, J. Geophys. Res.-Atmos., 126, e2019JD032344, https://doi.org/10.1029/2019JD032344, 2020.
Zsebeházi, G. and Szépszó, G.: Modeling the urban climate of Budapest using the SURFEX land surface model driven by the ALADIN-Climate regional climate model results, Időjárás, 124, 191–207, https://doi.org/10.28974/idojaras.2020.2.3, 2020.