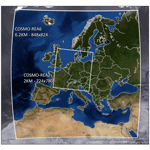
Regional atmospheric reanalysis activities at Deutscher Wetterdienst: review of evaluation results and application examples with a focus on renewable energy
Deborah Niermann
Michael Borsche
Stephanie Fiedler
Jan Keller
Roland Potthast
Thomas Rösch
Thomas Spangehl
Birger Tinz
Based on the numerical weather prediction model COSMO of Germany's national meteorological service (Deutscher Wetterdienst, DWD), regional reanalysis datasets have been developed with grid spacing of up to 2 km. This development started as a fundamental research activity within the Hans-Ertel-Centre for Weather Research (HErZ) at the University of Bonn and the University of Cologne. Today, COSMO reanalyses are an established product of the DWD and have been widely used in applications on European and national German level. Successful applications of COSMO reanalyses include renewable energy assessments as well as meteorological risk estimates. The COSMO reanalysis datasets are now publicly available and provide spatio-temporal consistent data of atmospheric parameters covering both near-surface conditions and vertical profiles. This article reviews the status of the COSMO reanalyses, including evaluation results and applications. In many studies, evaluation of the COSMO reanalyses point to an overall good quality and often an added value compared to different contemporary global reanalysis datasets. We further outline current plans for the further development and application of regional reanalyses in the HErZ research group Cologne/Bonn in collaboration with the DWD.
- Article
(2105 KB) - Full-text XML
- BibTeX
- EndNote
Germany's national meteorological service (Deutscher Wetterdienst, DWD hereafter) is responsible for monitoring climate in Germany. Traditionally, its approach was based on gridded products that are generated by interpolating observations from DWD's network of weather stations (e.g., Kaspar et al., 2013). However, this approach is restricted to near-surface variables and directly observed parameters. New applications require additional parameters or information at locations where long-term observations have not been taken. One such example is renewable energy, where wind speed estimates are needed at hub-heights of modern wind turbines (100 m and above). Such spatial estimates cannot be reasonably derived from existing observation networks.
Atmospheric model-based reanalyses are a methodology to generate consistent three-dimensional time-series datasets of the atmospheric state. In this respect, global reanalyses are an established and frequently used source of information for a wide range of applications. These data are typically generated by a global numerical weather prediction (NWP) model and a corresponding data assimilation system using historic observational data. At some research centers several generations of global reanalyses have been produced during the last decades (e.g., Gibson et al., 1997; Dee et al., 2011, or Hersbach et al., 2020 as selected examples for activities of the European Centre for Medium-Range Weather Forecasts (ECMWF)). Their spatial resolution has been continuously increased over the years, but is still too coarse for some applications (see results of the user survey described by Gregow et al., 2016).
During the last 15 years, efforts towards and implementations of regional reanalyses have been increasingly popular. A regional reanalysis uses a limited-area NWP model, driven at the lateral boundaries with conditions obtained from global reanalyses and constrained by the assimilation of observational data within its regional domain. Regional reanalyses have been developed for several regions in the world, e.g., the NCEP North American Regional Reanalysis (NARR; Mesinger et al., 2006), the Arctic System Reanalysis (ASR v2; Bromwich et al., 2018) and the Bureau of Meteorology Atmospheric high-resolution Regional Reanalysis for Australia (BARRA v1.0; Su et al., 2019).
In Europe, regional reanalyses have been produced by a number of national meteorological services, some of them covering the European continent, some smaller parts of Europe, e.g. Ireland (Gleeson et al., 2017) or Italy (Bonanno et al., 2019). A high-resolution regional reanalysis for Europe is being produced within the Copernicus Climate Change Service (CERRA – Copernicus European Regional ReAnalysis, Schimanke et al., 2019). Likewise, DWD's regional NWP-model COSMO (Schättler et al., 2011) has been used to develop a regional reanalysis for Europe (Bollmeyer et al., 2015).
The regional reanalysis developments at DWD started with the launch of the Hans-Ertel-Centre for Weather Research (”Hans-Ertel-Zentrum für Wetterforschung”, HErZ hereafter). HErZ was founded with the aim to improve the connection between fundamental meteorological research and teaching at German universities and atmospheric research institutes with the needs of DWD (Simmer et al., 2016). Another goal is to facilitate the transfer of fundamental research into operations. Following an open call, five priority research areas were established after review by an international panel of experts.
The Universities of Bonn and Cologne were successful with their joint proposal on the priority research area “Climate Monitoring and Diagnostics”. The group started to develop the first regional reanalysis based on DWD's NWP model systems in 2011 in close cooperation with DWD. After initial discussions, two configurations were implemented within the first phase of HErZ: A regional reanalysis for Europe covering the European CORDEX-domain with a 6.2 km horizontal resolution (COSMO-REA6; Bollmeyer et al., 2015), and a 2 km reanalysis for Central Europe (COSMO-REA2; Wahl et al., 2017). The European CORDEX domain (CoOrdinated Regional Downscaling Experiment; Kotlarski et al., 2014) was chosen for the former reanalysis to provide a dataset that is consistent with the configuration used in the regional climate modelling community. The COSMO-REA6 system was handed over to DWD in 2015 and regularly updated afterwards. Recently the updates came to a halt with the end of the availability of ERA-Interim (Dee et al., 2011), the global reanalysis that provided the lateral boundary conditions.
At around the same time, other national meteorological services in Europe also intensified their efforts to develop regional reanalysis systems. A joint proposal of a consortium including DWD and the HErZ group lead to the successful implementation of the EU-funded research project UERRA (Uncertainties in Ensembles of Regional ReAnalysis) that allowed for the assessment and comparison of the quality of individual systems (Borsche et al., 2015). With regard to HErZ and DWD efforts, an ensemble reanalysis at 12 km resolution was developed (COSMO-ENS-REA12; Bach et al., 2016) in addition to the two existing COSMO-based reanalyses. It was produced for a test period covering five years (2006—2010). Table 1 provides an overview of the main features of the three COSMO reanalysis datasets. Figure 1 illustrates the model domains.
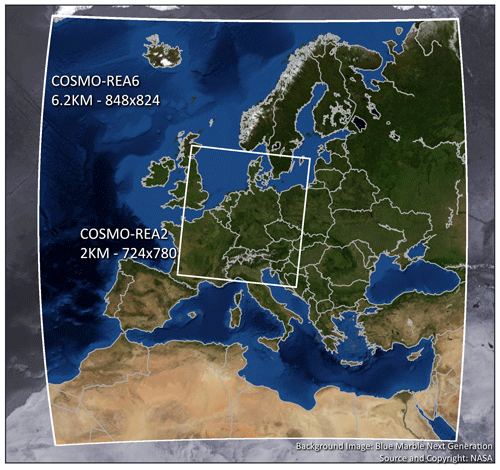
Figure 1Model domains of COSMO-REA6 and COSMO-REA2 (Land Cover Map: Blue Marble Next Generation, © NASA).
The UERRA project led to various conclusions on the quality of the specific datasets (see Sect. 2). One important aim for all producers of regional reanalyses is to demonstrate an added value compared to global reanalyses which are used as boundary conditions (e.g. Kaiser-Weiss et al., 2019). Because of the high user interest in parameters related to renewable energy, UERRA focused in particular on the evaluation of these parameters (e.g. Niermann et al., 2019).
In 2017, DWD introduced an open data policy based on a new formulation of the act that regulates the duties of DWD (Kaspar et al., 2019b). This has led to a wide usage of the COSMO reanalysis data, also outside of DWD and HErZ. This article aims to review these applications with a particular focus on the evaluation of COSMO reanalyses.
Several applications of the COSMO reanalyses have been published in the scientific literature. The majority of these are applications in the energy sector. For the energy sector several parameters are of particular interest, mainly wind speed profiles in the lower troposphere and surface solar radiation. Consequently, these variables have been extensively evaluated using observational data. The existing publications further show different ways to use the data. For surface solar radiation, other areal covering datasets exist (based on satellite or ground-based measurements). Authors therefore often start with an assessment of the quality in comparison with such observations. For wind related applications, less alternatives exist especially so at wind turbine hub heights. Therefore, the majority of these papers focus on the utilization of the reanalysis data in their applications.
Some energy related studies also use further reanalysis variables that influence energy production. An example is the use of wind gust data at 10 m above ground to investigate the effect of mechanical loads on PV modules (Camus et al., 2019). Moreover, the efficiency of photovoltaic (PV) modules is temperature-dependent and therefore influenced by temperature and wind (Kaspar et al., 2019a).
In order to provide an overview of the evaluations and applications of the COSMO regional reanalyses by DWD, HErZ and third parties, this paper is structured as follows: We review the existing evaluation results in Sect. 2 and the past applications of COSMO reanalyses in Sect. 3. In the concluding summary section, we provide a short outlook on the next generation of regional reanalyses as well as future directions for applications for renewable energies. These developments go hand-in-hand with the new NWP model system of DWD.
One specific challenge in the evaluation of reanalyses is that a large set of available observations is already used in the data assimilation. These observations can therefore not be used as independent information in evaluation efforts. However, various evaluations with independent observations have been performed for the COSMO reanalyses, often with a focus on the energy-related parameters wind and surface solar radiation.
2.1 Initial evaluations during development
During the setup and implementation of COSMO-REA6, several evaluations with independent measurements have been performed for a one-year test period as well as a comparison to an identical model simulation but without data assimilation (dynamical downscaling). In Bollmeyer et al. (2015), the development and configuration details of COSMO-REA6 are described. Results show that (a) the higher spatio-temporal resolution of the regional reanalysis leads to better representation of local extreme events and (b) compared to dynamical downscaling the regional reanalysis leads to an improved coherence with independent observations. In contrast to global reanalyses, the occurrence of heavy precipitation events is considerably better captured by the regional reanalysis and dynamical downscaling.
Wahl et al. (2017) described the development of the 2 km reanalysis for Central Europe (COSMO-REA2) where in addition to conventional observations, radar-derived rain rates were assimilated using a latent heat nudging scheme. The authors show an improved representation of local precipitation over Germany compared to coarser gridded European and global reanalyses. Based on a systematic verification using rain gauge data they illustrate the added value on different time scales: On monthly to annual time scales better estimates of the spatial variability of precipitation patterns are achieved compared to coarser reanalyses. On hourly to daily time scales, COSMO-REA2 substantially improves the representation of local precipitation in two ways: (a) An enhanced representation of observed frequencies of local precipitation, especially for high precipitation events, which is explained by the higher spatial resolution. (b) The assimilation of radar data largely improves the spatial and temporal coherence between COSMO-REA2 and local observations. In summary, the authors conclude that the combination of both contributions lead to reanalysed precipitation values which are comparable to point observations.
Bach et al. (2016) described the development of the 12 km probabilistic regional reanalysis system COSMO-ENS-REA12, where the perturbed atmospheric conventional observations were assimilated to generate a 20-member ensemble reanalysis. They assessed the representation of precipitation for selected months in a pilot study and showed that high grid resolution leads to an added value, particularly at higher precipitation thresholds, in comparison to the operational ECMWF-Ensemble Prediction System (ECMWF-EPS).
2.2 Evaluation of wind speed
Kaiser-Weiss et al. (2019) compared COSMO-REA6 with other European regional reanalyses (by the UERRA consortium) and the global reanalysis ERA-Interim with respect to the representation of wind speeds. By comparing with measurements from wind masts over Germany and the Netherlands they concluded, that for wind speeds at heights relevant for wind energy, the regional reanalyses can provide additional information at higher resolution horizontally, vertically, and in time, adding value to global reanalyses. Especially for the FINO1 and FINO2 masts in the North Sea and Baltic Sea they showed an advantage in the correlation for all European reanalyses compared to the global reanalysis.
Frank et al. (2020b) used four wind towers (Falkenberg, Billwerder and Karlsruhe in Germany; Cabauw in the Netherlands) to investigate the added value of COSMO-REA6 and COSMO-REA2 over global reanalyses. They showed that both perform mostly significantly better but at least similarly to global reanalyses. The COSMO reanalyses demonstrated significant improvements over global reanalyses especially in the marginal distributions (i.e., time-independent statistics), e.g. in the most extreme temporal wind changes (ramp rates) at typical hub-heights. The largest improvements can be found in biases, mean absolute errors, and correlations close to ground and in areas with complex terrain.
Good agreement in the wind speed histograms (including mean, median, 1st/99th percentile value) at hourly resolution of COSMO-REA6 with mast measurements in Falkenberg (Germany, close to DWD's observatory Linbenberg), Cabauw (the Netherlands), FINO1 (North Sea) and FINO2 (Baltic Sea) was also shown by Borsche et al. (2016, 2020). However, Borsche et al. (2016) also revealed shortcomings in the representation of the diurnal cycle at a height of 100 m.
Mast measurements are available only at a few locations. Kaiser-Weiss et al. (2015) compared regional and global reanalyses near-surface winds from the much denser network of regular meteorological station observations over Germany (some of them used in the data assimilation of COSMO-REA6). They showed that for the majority of the stations, the Weibull parameters of the daily mean wind speed frequency distribution matched well with the ones derived from the reanalysis fields. High correlations (reaching values larger than 0.9) can be found between stations and reanalysis monthly mean wind speeds all over Germany. Generally, the correlation between the better resolved COSMO-REA6 wind fields and station observations is highest, for both assimilated and non-assimilated, i.e., independent, observations. Niermann et al. (2018, 2019) also evaluated the wind speed from various European reanalyses by comparing against near-surface wind speed of these German stations. They showed for the 10 m wind speed that the maximum peak correlation between observations and regional reanalyses is reached at a weekly timescale. Also at other temporal resolutions the regional reanalyses show better correlations than ERA-Interim. It was further shown that the agreement of the frequency distributions might differ significantly between regional reanalysis and station measurements when the height of the model grid cells differs significantly from the station height.
As global reanalyses also undergo continuous developments, relevant progress has been made for these, e.g. in terms of higher horizontal resolutions. ERA5 with a 30 km grid resolution (Hersbach et al., 2020) has become available as the successor of ERA-Interim (at 80 km grid resolution). The comparison of near-surface wind speed from Niermann et al. (2019) has therefore been reproduced with ERA5 being included. Figure 2 shows that there is a significant improvement for ERA5 over ERA-Interim in the correlation with wind measurements recorded at German stations, but COSMO-REA6 still has an advantage compared to both global reanalyses.
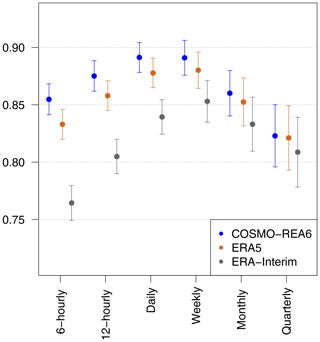
Figure 2Correlation of wind speed of various reanalysis datasets with near-surface wind speed measurements recorded by German stations: Time dependence of the Pearson correlation coefficient averaged over 128 German locations above 100 m station height for COSMO-REA6 and the two global reanalyses ERA5 and ERA-Interim derived from the time period January 1995–August 2019. Averages are based on reanalysis and observational data sampled every hour, except for the 6 h ERA-Interim analysis. The error bars mark the 95 % confidence interval extracted from the sample of stations. The figure was produced in analogy to Fig. 1b of Niermann et al. (2019), but for a longer period and additionally contains ERA5. Locations above 100 m station height have been chosen, because wind measurements from these stations have not been assimilated in the production of COSMO-REA6.
Ganske (2020) used COSMO-REA6 together with data from stations on German islands for the evaluation of wind speed and direction in regional climate simulations for German coastal regions. These comparisons show a high correlation of wind speed from COSMO-REA6 with these observations (e.g., Fig. 7 in Ganske, 2020).
Fery and Tinz (2020) evaluated single storm events by comparing COSMO-REA6 with observations at the FINO-masts in the North Sea. They found very good agreement in wind speed, wind direction and surface pressure for the selected examples and concluded that COSMO-REA6 is able to realistically reproduce historical storms.
Jourdier (2020) compared data from various reanalyses with wind power production data over France. In this comparison, COSMO-REA6 had smaller biases than for example the global reanalysis MERRA-2 (Modern-Era Retrospective Analysis for Research and Applications, Version 2, Gelaro et al., 2017) or the New European Wind Atlas “NEWA” (Hahmann et al., 2020) datasets and better skill in complex areas than ERA5.
Pickering et al. (2020) assessed the capability of COSMO-REA2 and COSMO-REA6 to reproduce historical measured data from weather stations, hub height anemometers, and wind turbine electricity generation across Switzerland. They concluded for both reanalyses that they are insufficient to reproduce site-specific wind speeds in Switzerland's complex terrain, but found that mountain-valley breezes, orographic channelling, and variability imposed by European-scale weather regimes are represented by COSMO-REA2. They discovered situations of wind electricity generation in regions of Switzerland which are anti-correlated with neighbouring European countries. Yet, they conclude that higher-resolution reanalyses are necessary to accurately understand the local variability of renewable generation in complex terrain, like the Swiss Alps.
Ramirez Camargo et al. (2019a) assessed wind and radiation from COSMO-REA6 and COSMO-REA2 using data from 28 weather stations of the Bavarian agro-meteorological network and the Czech Hydrometeorological Institute. The purpose of their study was to assess the quality of variables relevant for modelling small-scale renewable energy systems and to assess the advantage over coarser global reanalyses. They used several accuracy indicators for hourly data and showed that: (a) indicators such as the Pearson's correlation coefficient in some cases reach values above 0.82 for wind speeds and above 0.92 for global horizontal irradiance and (b) the mean bias error is consistently better than ±9.3 W m−2 for the full set of solar irradiance data, ±25.1 W m−2 for only day-time irradiance data and is with a few exceptions lower than ±1 m s−1 for wind speed data. They generally conclude that for these parameters in their study region, results are in most cases comparable or better than for global reanalysis datasets.
In summary, the studies consistently conclude that COSMO-REA6 provides wind fields with added value in comparison to global reanalyses. Some issues remain, especially shortcomings in the diurnal cycle at around 100 m. This deficit in the COSMO-model and potential for improvement have been discussed in detail by Heppelmann et al. (2017) and the issue might therefore be improved in the next generations of regional reanalysis datasets.
2.3 Evaluation of radiation
Niermann et al. (2019) used two satellite-based surface radiation datasets (SARAH-2: Surface Solar Radiation Data Set – Heliosat – Edition 2 and HelioMont) for the evaluation of monthly mean solar irradiance. The comparison of the solar irradiance of the reanalyses with SARAH-2 reveals a negative bias for the COSMO-REA6, while other European regional reanalysis products show an overestimation of solar irradiance. Over the Alpine region, all regional reanalyses show high positive anomalies against the European-wide SARAH-2 dataset. These anomalies vanish when HelioMont is used, which is a high-quality satellite reference dataset specifically developed for the Alpine region (Stöckli, 2003).
Frank et al. (2018) evaluated the COSMO-REA6 dataset using quality-controlled measurements of the Baseline Surface Radiation Network (BSRN) and found systematic biases for specific conditions: a systematic underestimation under clear sky conditions (caused by the aerosol climatology) and an overestimation during cloudy conditions (caused by an underestimation of the optical depth of clouds). They developed a post-processing scheme to correct for these systematic deficits and showed that the post-processed dataset (COSMO-REA6pp) clearly outperforms the global reanalyses ERA-Interim and MERRA-2 with a lower bias and mean absolute error. The underestimation of radiation under clear sky conditions is also addressed by Urraca et al. (2018b). They evaluated several solar radiation datasets, including COSMO-REA6 by comparing station data, global reanalysis datasets and satellite data. Typically, ground-based measurements are used as reference, but the authors also show relevant quality limitations for those, e.g. in case of poorly maintained and incorrectly calibrated photodiodes.
Urraca et al. (2018a) examined the surface irradiance of COSMO-REA6 and ERA5 against stations in Europe, and two satellite-derived products (NSRDB and SARAH). They conclude that ERA5 and COSMO-REA6 have reduced the gap between reanalysis and satellite-based data. They also conclude that COSMO-REA6 underestimates surface radiation at most stations with the largest deviations under clear-sky conditions. They show that northward of 45∘ N the magnitude of the biases and absolute errors of COSMO-REA6 are similar to ERA5, while it outperforms ERA5 in coastal areas due to the higher spatial resolution. They suggest that satellite-based data are the preferred choice, when available, but suggest that ERA5 is an alternative for situations in which satellite-based data are missing (polar regions and gaps in time series) while COSMO-REA6 could complement ERA5 in Central and Northern Europe mitigating the limitations of ERA5 in coastal areas.
2.4 Evaluation of other parameters
Scherrer (2020) presented an evaluation of temperature fields and trends in the Alps in recent decades. He compared the temperature fields from COSMO-REA6, ERA5 and two other regional reanalyses with Swiss stations in order to evaluate the suitability of datasets for temperature monitoring. For high altitudes, he showed that the regional reanalysis COSMO-REA6 outperforms the other datasets for the Alpine setting at high elevations, especially in winter, indicating the benefit of the higher spatial resolution compared to ERA5.
Kaiser-Weiss et al. (2019) compared precipitation fields of the regional reanalyses from the UERRA ensemble with high-resolution gridded datasets from the Alpine region and Fennoscandia. They concluded that the regional reanalyses add value to the global reanalysis ERA-Interim because of higher resolution of topography and that the COSMO reanalyses (COSMO-REA6, COSMO-ENS-REA12) show the best performance in the two regions.
Lockhoff et al. (2019) evaluated several daily precipitation products over western and central Europe with a focus on daily extremes. They analysed one gauge-based, three satellite-based, and two reanalysis-based products (COSMO-REA6, HIRLAM) using high-density rain gauge observations as a reference. They showed that the regional reanalysis datasets are well able to represent the precipitation characteristics and that the regional reanalysis COSMO-REA6 shows the largest skill among the non-station-based datasets at all analysed scales for the summer and winter seasons (based on the fractions skill score, used to assess the skill of the candidate datasets to represent the occurrences of extreme precipitation compared to the reference).
Steinke et al. (2019) evaluated the ability of COSMO-REA6 and COSMO-REA2 to represent integrated water vapour (IWV) relative to ERA-Interim with reference data from 133 ground-based Global Positioning System (GPS) stations distributed over Germany and revealed a clear superiority of the COSMO-REA products for providing instantaneous IWV. The improvement is visible on the daily and even monthly scale and is attributed to a better representation of surface heterogeneity and boundary-layer processes on small scales.
Springer et al. (2017) used total water storage estimates from the Gravity Recovery and Climate Experiment (GRACE) mission in combination with discharge data for assessing the closure of the water budget in COSMO-REA6 in comparison to the global reanalyses ERA-Interim and MERRA-2 and two observation-based datasets (Global Precipitation Climatology Centre (GPCC), Global Land Evaporation Amsterdam Model (GLEAM)). They found that for most catchments, COSMO-REA6 closes the water budget within the error estimates, whereas the global reanalyses underestimate net precipitation (P-E) by up to 20 mm month−1.
In summary, the evaluation results cover a spectrum of variables and therefore suggest that COSMO-REA6 is able to provide a realistic representation of the atmospheric state for a range of parameters.
In addition to the aforementioned papers with evaluation results, the COSMO reanalyses have been used in several applications. While the majority of these applications have been implemented in the context of renewable energies, some other examples were published for other fields of application. In the following two sections, we provide an overview of energy-related as well as other applications.
3.1 Applications related to renewable energies
Henckes et al. (2018) discussed the potential benefit of long-term high resolution wind data from COSMO-REA6 for electricity system analysis and identified advantages of the high-resolution dataset compared to previous studies. Based on COSMO-REA6 they identified a strong variation in annual wind production (variation of up to 14 % for Germany) and illustrated the potential benefit from electricity exchange with neighbouring countries in low wind conditions (e.g., for Germany, they found that in 81 % of the low wind situations balancing effects in wind power production with neighbouring countries can be expected). They argue that such results are highly relevant for investigations on the level of secured capacity or to identify optimal power transmission capacities within energy market modelling.
Germer and Kleidon (2019) used COSMO-REA6 to evaluate whether wind turbines in Germany have generated electricity as would be expected from the prevailing wind conditions in 2000–2014. They used German monthly energy yield data on a turbine basis and related those to energy yield predicted on the basis of wind speed and air density calculated from the reanalysis dataset COSMO-REA6. They applied a statistical model and quantified factors that reduced actual compared to ideal yields. They concluded that the combination of ideal yields predicted from wind conditions with observed yields is suitable to derive realistic estimates of wind energy generation as well as realistic resource potentials.
Kaspar et al. (2019a) used wind data from COSMO-REA6 in combination with satellite-derived surface radiation to assess balancing effects and shortfall risks of photovoltaics and wind energy in Germany and Europe. The efficiency of photovoltaic energy production depends on module temperature, therefore the calculation also requires information on temperature and wind speed which was as well taken from COSMO-REA6 in their study. They concluded that on average, the seasonal cycles of PV and wind power production complement each other in Germany as well as in Europe. The authors analysed the frequency of events with a risk of low electricity generation under different assumptions. Compared to their reference case (wind energy over German land areas), their results illustrate that the number of low production events is reduced when offshore wind energy in Germany's Exclusive Economic Zone is included in the analysis, or when a combined system of PV and wind energy is considered. Likewise, a European-wide analysis leads to a distinct reduction of such events. Frank et al. (2020c) also used COSMO-REA6 for an analysis of cross-country balancing effects of wind and photovoltaics.
Becker and Thrän (2018) used COSMO-REA6 in a study on optimal siting of wind farms in wind energy dominated power systems where the optimal wind farm site is the result of optimized wind speed correlations with other wind farms. A case study of the model's applicability was performed for Germany. The results indicate that a full market integration of renewable energy generators will space out new wind farms more evenly. The authors conclude that under such assumptions, wind farms can economically benefit from the non-simultaneity of wind speed.
Wind data from COSMO-REA6 is also used in the context of the “Site Development Plan 2019 for the German North Sea and Baltic Sea” (BSH, 2020a (German), b (English); Tinz et al., 2019). The Site Development Plan determines areas for new installations of offshore wind turbines within the German Exclusive Economic Zone of the North and Baltic Sea (see Fig. 3) for the period 2026 until at least 2030 (on the basis of the German Wind Energy at Sea Act).
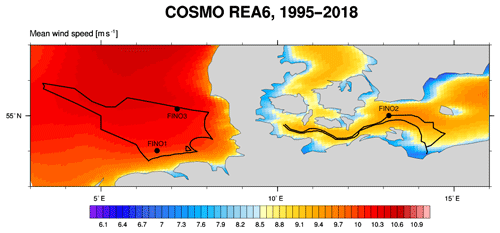
Figure 3COSMO-REA6 average wind speed 1995–2018 in the German Exclusive Economic Zone (EEZ) in the North Sea and Baltic Sea at 116 m height (level 4 of COSMO-REA6 above ground). The boundaries of the EEZ and the locations of the FINO (1, 2, 3) measurement sites are also shown.
Peter and Wagner (2018) evaluated the capacity value of variable renewable energy, i.e., its contribution to security of supply. They developed a framework to determine the capacity value for electricity markets, which accounts for balancing effects due to the spatial distribution of generation capacities and interconnectors. Based on COSMO-REA6 data, they applied the framework to wind power in Europe and found that wind power can substantially contribute to security of supply in a decarbonized European electricity system in 2050. They argue that ignoring the temporal and spatial heterogeneity of the contribution of wind power to security of supply would lead to inefficient levels of dispatchable back-up capacity.
Camus et al. (2019) used COSMO-REA2 data to demonstrate the capability of meteorological reanalysis data for estimating mechanical stresses due to wind gusts on photovoltaic modules.
Steinheuer and Friederichs (2020) presented a statistical post-processing method to derive a conditional distribution for hourly wind gusts as a function of height. The conditioning variables are taken from COSMO-REA6. The authors showed that the post-processing model with a Legendre approximation is able to provide reliable predictions of gusts' statistics at non-observed intermediate levels.
Jensen and Pinson (2017) describe the development of “Renewable Energy Europe (RE-Europe)”, a large-scale dataset for modelling a highly renewable European electricity system. The dataset was assembled from several components: the power network, conventional generators, as well as renewable energy generation and electric power demand. For the weather-dependent signals they used COSMO-REA6 and the ECMWF deterministic forecasts to derive wind and solar power available at each node using simple physical models of wind turbine and solar panel power curves.
Ramirez Camargo et al. (2018) used radiation and temperature data from COSMO-REA6 to analyse requirements for electricity self-sufficiency of single-family houses based on photovoltaics and battery systems in Germany and the Czech Republic within the project “CrossEnergy: energy infrastructure – future perspectives for a region in change”. The study presents a methodology for generating maps indicating minimum battery and photovoltaic sizes for self-sufficient single-family houses in that region. Ramirez Camargo et al. (2019b) evaluated how and at what cost electricity demand of residential users across Germany and the Czech Republic could be covered by hybrid renewable energy generation systems consisting of photovoltaics, micro-generation wind turbines and batteries. COSMO-REA6 data (together with satellite data for snow coverage) were used to calculate necessary system sizes over a large area by simultaneously accounting for the temporal variability of renewable energy.
In the EU-project CLIM2POWER (“Translating climate data into power plant operational guidance”) COSMO-REA6 was chosen to serve as consistent local meteorological information in combination with an empirical-statistical downscaling technique (EPISODES: Kreienkamp et al., 2019) to transform global information from a seasonal forecasting system to the regional scale. The spatial resolution of ∼6 km is suitable for hydro modelling and the dataset also provides other energy relevant variables like wind speed at hub height (Ostermöller et al., 2019).
COSMO-REA6 was also applied in combination with satellite data to assess potential PV yield within the “Network of Experts” of the German Federal Ministry of Transport and Digital Infrastructure (BMVI), topic 5: “Enhanced development of renewable energy in transport and infrastructure”. The partners within the network evaluated the potential of renewable energies alongside the German transport infrastructure (i.e., railways, motorways, waterways, including associated buildings and other elements of the infrastructure) (BMVI-Expertennetzwerk, 2020).
COSMO-REA6 was used as one alternative dataset in PV-GIS Version 5 (Photovoltaic Geographical Information System), an online web application of the EU Joint Research Centre (JRC) for site-specific solar resource assessment (PV-GIS, 2020). Their primary datasets are satellite-based radiation datasets, but they are not available for all regions and are therefore complemented with reanalysis data (Urraca et al., 2018a).
3.2 Further application examples
Naz et al. (2020) used several meteorological variables from COSMO-REA6 to provide atmospheric forcing data (such as solar radiation, temperature, pressure, near-surface wind speed, specific humidity and precipitation rate) to produce a high-resolution spatially and temporally consistent surface soil moisture reanalysis (ESSMRA) dataset (3 km, daily) over Europe from a land surface data assimilation system. The dataset provides long-term (2000–2015) daily surface soil moisture at a high spatio-temporal resolution and is considered to be beneficial for many hydrological applications over regional and continental scales. The methodology is described in Naz et al. (2019).
Bonanno et al. (2019) use COSMO-REA6 and ERA5 to verify the MEteorological Reanalysis Italian DAtaset (MERIDA). MERIDA consists of a dynamical downscaling of the ERA5 global reanalysis fields with 7 km resolution using the mesoscale model WRF. Additionally, 2 m temperature and precipitation fields are downscaled on a regular 4 km grid resolution, with an optimal interpolation technique in combination with station data. In the verification against ERA5 and COSMO-REA6, MERIDA showed comparable or better results in the reconstruction of 2 m temperature and precipitation. Clear improvement (with respect to MERIDA) was obtained for the post-processed MERIDA output. The authors conclude that for the other models, there is a decline in performance in the mountainous areas, where there are likely to be more significant deviations between the real and model orographic height.
Keller et al. (2017) suggest a statistical downscaling approach for precipitation based on the “analog ensemble” (AnEn) method. The approach was implemented and tuned on the basis of a set of over 700 rain gauges with 6-hourly measurements for Germany and predictors from COSMO-REA6. The authors showed that with respect to deterministic quality, the results show that AnEn is able to outperform the reanalysis itself depending on location and precipitation intensity.
Paeth et al. (2017) present a physical model approach for orographic precipitation based on linear theory and combined with a stochastic weather generator. The derived precipitation fields are spatially and temporally compared with station data from a very dense meteorological network and with COSMO-REA6. As an additional outcome, they concluded that COSMO-REA6 shows excellent performance for precipitation over the Harz region (a German low mountain range), i.e., a very realistic simulation of spatial and temporal precipitation variability.
Carbajal Henken et al. (2020) assessed sampling effects on satellite-derived Integrated Water Vapor (IWV) datasets using Global Positioning System (GPS) measurements in Germany. A quality check was applied to the GPS data, also with the help of comparisons with COSMO-REA2. The authors showed that IWV values are dominated by the overall retrieval bias. Sampling only marginally affects the mean values, but can lead to significant changes in the frequency distributions.
Rasquin et al. (2020) investigated the role of the coastal bathymetry for the response of tidal dynamics to mean sea level rise in the German Bight. The atmospheric forcing (wind at 10 m and atmospheric surface pressure) for the numerical hydrodynamic model was taken from COSMO-REA6. They showed that the resolution of the bathymetry especially in flat intertidal areas plays a crucial role for modelling the impact of mean sea level rise. Wachler et al. (2020) used the same model system to assess the tidal response to sea level rise and bathymetric changes.
Hinrichs et al. (2019) developed the Baltic and North Seas Climatology (BNSC), a gridded dataset of atmospheric and hydrographic parameters which was calculated solely from marine in situ observations. The authors used COSMO-REA6 and two global reanalyses to evaluate the quality of the atmospheric parameters of the dataset. The comparison of sea-level pressure of BNSC and COSMO-REA6 for 1995–2015 showed mean deviations between −1 to 1 hPa.
The project KISP (“KI gestützte Schadstoffprognose zur intelligenten Verkehrssteuerung“) applied Artificial Intelligence methods in combination with data from COSMO-REA6 and local air pollution measurements from selected German cities to develop NO2-forecasts for intelligent traffic management (WIK, 2019). Correlations between meteorological parameters and local NO2 concentration were found, but it was also shown that the results depend on the category of the station type (Enercast, 2019).
The active development of a regional reanalysis system based on DWD's numerical weather prediction system COSMO started in 2011 within the Hans-Ertel-Centre for Weather Research at the Universities of Bonn and Cologne in cooperation with DWD. Three main configurations have been developed: a 6 km reanalysis covering Europe, a 2 km reanalysis covering Central Europe and a 12 km ensemble version also covering Europe. The datasets have been made publicly available and have been used in a variety of applications. Various parameters have been evaluated and in many cases added value over global reanalysis datasets has been shown. The results suggest that COSMO-REA6 provides a realistic representation of the atmospheric state across parameters. The majority of applications in the scientific literature are related to renewable energy and illustrate thereby the high relevance of high-resolution meteorological datasets for such applications.
As the existing COSMO-based reanalyses were developed several years ago, the COSMO model versions used to produce the datasets are now quite old. Therefore, efforts at DWD are currently underway to use a more recent COSMO version to produce a new (and extended) COSMO-reanalysis dataset.
Further, the NWP activities of DWD have moved to a completely new model system (ICON; ICOsahedral Non-hydrostatic, see Zängl et al., 2015). Moreover, significant progress has also been made in the methodologies for data assimilation. The existing reanalyses are based on a nudging of observations scheme (Schraff and Hess, 2003), while the current NWP system at DWD is based on a Local Ensemble Transform Kalman Filter (LETKF), which has led to significant improvements in forecast skill (Schraff et al., 2016; Potthast et al., 2019). To benefit from these improvements in the reanalysis context, DWD started work on preparing the next generation of reanalysis based on the new model and data assimilation systems.
In addition, efforts at the HErZ group in Bonn and Cologne will continue to focus on the development, evaluation and exploitation of regional reanalysis. Current research is underway to assess the potential for future regional reanalysis at horizontal resolutions of 1 km and below with DWD's new ICON limited area model (ICON-LAM) model and data assimilation chain. The focus is on the potential improvements in the representation of land surface heterogeneities and the effect on reproducing urban environments – a shortcoming of all current reanalyses. This development aims to further push the boundaries in climate monitoring through fundamental research on high-resolution regional reanalysis.
Further HErZ efforts aim at implementing a boundary layer reanalysis (analogous to a surface reanalysis approach) where existing reanalysis data are used as a background for a post-processing data assimilation step in which observations are assimilated that were not used in the production of the reanalysis. This is expected to produce a consistent long-term three-dimensional dataset which is closer to the true state of the atmosphere compared to the driving COSMO reanalysis.
The HErZ group will also continue to exploit the COSMO family of regional reanalyses for renewable energy applications. A focus is on using this data for answering current questions regarding the planned energy transition in Europe. Special interests include economic estimates of regional energy self-sufficiency, the implication of meteorological extremes for the reliability of the energy production, and the usage of COSMO reanalyses in models for energy plants and energy systems of different complexity. Further work includes improvements of wind gusts at levels above 10 m using statistical modelling and exploiting new scanning techniques for ground-based remote sensing instruments. In this context, the new reanalysis system will also be applied for testing the usefulness of assimilating observations from new measurement instruments, estimating the impact of changes in the observation network, and synthesizing various measurements from field campaigns such as FESSTVal (“FESSTVaL (“Field Experiment on Submesoscale Spatio-Temporal Variability in Lindenberg”, http://fesstval.de/, last access: 3 July 2020, Wiesner et al., 2019) as a best estimate of the full 3D structure of the atmosphere.
The recently started BMBF-funded German research consortium ClimXtreme will focus on improved understanding of climate change and extreme events. In that context, the ability of the COSMO-reanalyses to represent extreme events will be further evaluated.
In the project FAIR (“User-friendly provision of climate and weather data”) improved user-friendly access to the COSMO reanalysis datasets will be developed in close cooperation with potential users. Within the project, also the use of third-party data to improve the reanalysis will be tested, e.g. power generation data of wind turbines or crowdsourced sensing data coming from individual smartphones (Frank et al., 2020a).
Selected parameters of the COSMO-REA6 regional reanalysis are publicly available via DWD's Climate Data Center: https://opendata.dwd.de/climate_environment/REA/ (DWD/HErZ, 2020). Selected parameters of the COSMO-REA2 regional reanalysis are publicly available via the Hans-Ertel-Centre for Weather Research/University of Bonn: https://reanalysis.meteo.uni-bonn.de/?Download_Data___COSMO-REA2 (HErZ, 2020a). The post-processed radiation dataset (COSMO-REA6pp) is also publicly available via the Hans-Ertel-Centre for Weather Research/University of Bonn: https://reanalysis.meteo.uni-bonn.de/?Derived_data_sets__Post-Processed_Radiation (HErZ, 2020b).
FK wrote the first draft of the manuscript. All authors contributed to the work and ideas presented here and commented on the manuscript. DN performed the updated comparison between COSMO-REA6, ERA-Interim and ERA5 (Fig. 2). JK produced Fig. 1. TS produced Fig. 3.
The authors declare that they have no conflict of interest.
This article is part of the special issue “19th EMS Annual Meeting: European Conference for Applied Meteorology and Climatology 2019”. It is a result of the EMS Annual Meeting: European Conference for Applied Meteorology and Climatology 2019, Lyngby, Denmark, 9–13 September 2019.
We are grateful for the contributions of all former team members in the HErZ group and at DWD. The HErZ group at Cologne/Bonn acknowledges the continuous support and fruitful collaboration with DWD. Two anonymous referees gave helpful comments on the manuscript.
This research has been supported by the BMVI Network of Experts, the BMVI mFund (project FAIR, grant no. 19F2103C) and the BMBF programme ClimXtreme (grant no. 01LP1904A). The development of the ICON-based reanalysis is supported by the DWD research program “Innovation in Applied Research and Development” (IAFE). The UERRA project was supported by the European Seventh Framework Programme (grant no. 607913).
This paper was edited by Eric Bazile and reviewed by two anonymous referees.
Bach, L., Schraff, C., Keller, J. D., and Hense, A.: Towards a probabilistic regional reanalysis system for Europe: Evaluation of precipitation from experiments, Tellus A, 68, 32209, https://doi.org/10.3402/tellusa.v68.32209, 2016.
Becker, R. and Thrän, D.: Optimal Siting of Wind Farms in Wind Energy Dominated Power Systems, Energies, 11, 978, https://doi.org/10.3390/en11040978, 2018.
BMVI-Expertennetzwerk: Einsatzpotenziale erneuerbarer Energien für Verkehr und Infrastruktur verstärkt erschließen – Ergebnisbericht des Themenfeldes 5 im BMVI-Expertennetzwerk für die Forschungsphase 2016–2019, Bundesministerium für Verkehr und digitale Infrastruktur (BMVI), Berlin, 2020.
Bollmeyer, C., Keller, J. D., Ohlwein, C., Wahl, S., Crewell, S., Friederichs, P., Hense, A., Keune, J., Kneifel, S., Pscheidt, I., Redl, S., and Steinke, S.: Towards a high-resolution regional reanalysis for the European CORDEX domain, Q. J. Roy. Meteor. Soc., 141, 1–15, https://doi.org/10.1002/qj.2486, 2015.
Bonanno, R., Lacavalla, M., and Sperati, S.: A new high-resolution Meteorological Reanalysis Italian Dataset: MERIDA, Q. J. R. Meteor. Soc., 145, 1756–1779, https://doi.org/10.1002/qj.3530, 2019.
Borsche, M., Kaiser-Weiss, A. K., Undén, P., and Kaspar, F.: Methodologies to characterize uncertainties in regional reanalyses, Adv. Sci. Res., 12, 207–218, https://doi.org/10.5194/asr-12-207-2015, 2015.
Borsche, M., Kaiser-Weiss, A. K., and Kaspar, F.: Wind speed variability between 10 and 116 m height from the regional reanalysis COSMO-REA6 compared to wind mast measurements over Northern Germany and the Netherlands, Adv. Sci. Res., 13, 151–161, https://doi.org/10.5194/asr-13-151-2016, 2016.
Borsche, M., Kaiser-Weiss, A. K., and Kaspar, F.: Corrigendum to “Wind speed variability between 10 and 116 m height from the regional reanalysis COSMO-REA6 compared to wind mast measurements over Northern Germany and the Netherlands”. Adv. Sci. Res., https://doi.org/10.5194/asr-13-151-2016-corrigendum, 2020.
Bromwich, D. H., Wilson, A. B., Bai, L., Liu, Z., Barlage, M., Shih, C. F., Maldonado, S., Hines, K. M., Wang, S. H., Woollen, J., Kuo, B., Lin, H. C., Wee, T. K., Serreze, M. C., and Walsh, J. E.: The Arctic system reanalysis, version 2, B. Am. Meteorol. Soc., 99, 805–828, https://doi.org/10.1175/BAMS-D-16-0215.1, 2018.
BSH: Flächenentwicklungsplan 2019 für die deutsche Nord- und Ostsee: Bundesamt für Seeschifffahrt und Hydrographie, 216 pp., Hamburg, https://www.bsh.de/DE/THEMEN/Offshore/Meeresfachplanung/Flaechenentwicklungsplan/flaechenentwicklungsplan_node.html, last access: 3 July 2020a.
BSH: Site Development Plan for the German North Sea and Baltic Sea – unofficial translation: Bundesamt für Seeschifffahrt und Hydrographie, 211 pp., Hamburg, available at: https://www.bsh.de/EN/TOPICS/Offshore/Sectoral_planning/Site_development_plan/site_development_plan_node.html, last access: 3 July 2020b.
Camus, C., Offermann, P., Weissmann, M., Buerhop, C., Hauch, J., and Brabec, C. J.: Site-specific assessment of mechanical loads on photovoltaic modules from meteorological reanalysis data, Sol. Energy, 188, 1134–1145, https://doi.org/10.1016/j.solener.2019.06.077, 2019.
Carbajal Henken, C., Dirks, L., Steinke, S., Diedrich, H., August, T., and Crewell, S.: Assessment of Sampling Effects on Various Satellite-Derived Integrated Water Vapor Datasets Using GPS Measurements in Germany as Reference, Remote Sensing, 12, 1170, https://doi.org/10.3390/rs12071170, 2020.
Dee, D., Uppala, S. M., Simmons, A. J., Berrisford, P., Poli, P., Kobayashi, S., Andrae, U., Balmaseda, M. A., Balsamo, G., Bauer, P., Bechtold, P., Beljaars, A. C. M., van de Berg, L., Bidlot, J., Bormann, N., Delsol, C., Dragani, R., Fuentes, M., Geer, A. J., Haimberger, L., Healy, S. B., Hersbach, H., Hólm, E. V., Isaksen, L., Kållberg, P., Köhler, M., Matricardi, M., McNally, A. P., Monge-Sanz, B. M., Morcrette, J.-J., Park, B.-K., Peubey, C., de Rosnay, P., Tavolato, C., Thépaut, J.-N., and Vitart, F.: The ERA-Interim reanalysis: configuration and performance of the data assimilation system, Q. J. Roy. Meteor. Soc., 137, 553–597, https://doi.org/10.1002/qj.828, 2011.
DWD/HErZ (Deutscher Wetterdienst – Climate Data Center/Hans-Ertel Centre for Weather Research): COSMO-REA6 regional reanalysis, available at: https://opendata.dwd.de/climate_environment/REA, last access: 7 March 2020.
Enercast: KISP – KI gestützte Schadstoffprognose, Kurzzusammenfassung, Kassel, Germany, 2019.
Fery, N. and Tinz, B.: EXTREMENESS-B - Analyse von Windfeldern, die extreme Sturmfluten verursachen können. 22. KFKI-Seminar, DSM Bremerhaven, http://www.kfki.de/files/kfki-seminare/0/22_Abstract_EXTREMENESS_Fery_Tinz.pdf, last access: 3 July 2020.
Frank, C. W., Kaspar, F., Keller, J. D., Adams, T., Felkers, M. S., Fischer, B., Handte, M., Marrón, P. J., Paulsen, H., Neteler, M., Schiewe, J., Schuchert, M., Wacker, R., and Figura, R.: FAIR: A project to realize a user-friendly exchange of open weather data, Adv. Sci. Res., in review, 2020a.
Frank, C. W., Pospichal, B., Wahl, S., Keller, J. D., Hense, A., and Crewell, S.: The added value of high resolution regional reanalyses for wind power applications, Renew. Energ., 148, 1094–1109, https://doi.org/10.1016/j.renene.2019.09.138, 2020b.
Frank, C. W., Fiedler, S., and Crewell, S.: Balancing potential of natural variability and extremes in photovoltaic and wind energy production for European countries, Renew. Energ., in review, 2020c.
Frank, C. W., Wahl, S., Keller, J. D., Pospichal, B., Hense, A., and Crewell, S.: Bias correction of a novel European reanalysis data set for solar energy applications, Sol. Energy, 164, 12–24, https://doi.org/10.1016/j.solener.2018.02.012, 2018.
Ganske, A.: Validation von Windfeldergebnissen auf See und an der Küste aus regionalen Gekoppelten Ozean Atmosphäre Klimamodellen. Meilenstein SP-104, BMVI-Expertennetzwerk Schwerpunkt Sturm, Bundesamt für Seeschifffahrt und Hydrographie. 20.6.2019: https://www.bsh.de/DE/PUBLIKATIONEN/_Anlagen/Downloads/Meer_und_Umwelt/Expertennetzwerk/Validation_Wind-auf-See.pdf, last access: 3 July 2020.
Gelaro, R., McCarty, W., Suárez, M. J., Todling, R., Molod, A., Takacs, L., Randles, C. A., Darmenov, A., Bosilovich, M. G., Reichle, R., Wargan, K., Lawrence Coy, L., Cullather, R., Draper, C., Akella, S., Buchard, V., Conaty, A., da Silva, A. M., Gu, W., Kim, G.-K., Koster, R., Lucchesi, R., Merkova, D., Nielsen, J. E., Partyka, G., Pawson, S., Putman, W., Rienecker, M., Schubert, S. D., Sienkiewicz, M., and Zhao, B.: The modern-era retrospective analysis for research and applications, version 2 (MERRA-2). J. Climate, 30, 5419–5454, https://doi.org/10.1175/JCLI-D-16-0758.1, 2017.
Germer, S. and Kleidon, A.: Have wind turbines in Germany generated electricity as would be expected from the prevailing wind conditions in 2000–2014?, PLoS ONE, 14, e0211028, https://doi.org/10.1371/journal.pone.0211028, 2019.
Gibson, J. K., Kållberg, P. W., Uppala, S., Hernandez, A., Nomura, A., and Serrano, E.: ERA description. ECMWF Re-Analysis Project Report Series 1, 72 pp., ECMWF, Shinfield Park, Reading, UK, https://www.ecmwf.int/node/9584 (last access: 3 July 2020), 1997.
Gleeson, E., Whelan, E., and Hanley, J.: Met Éireann high resolution reanalysis for Ireland, Adv. Sci. Res., 14, 49–61, https://doi.org/10.5194/asr-14-49-2017, 2017.
Gregow, H., Jylhä, K., Mäkelä, H. M., Aalto, J., Manninen, T., Karlsson, P., Kaiser-Weiss, A. K. , Kaspar, F., Poli, P., Tan, D. G. H., Obregon, A., and Su, Z.: Worldwide survey of awareness and needs concerning reanalyses and respondents views on climate services, B. Am. Meteorol. Soc., 97, 1461–1473, https://doi.org/10.1175/BAMS-D-14-00271.1, 2016.
Hahmann, A. N., Sile, T., Witha, B., Davis, N. N., Dörenkämper, M., Ezber, Y., García-Bustamante, E., González Rouco, J. F., Navarro, J., Olsen, B. T., and Söderberg, S.: The Making of the New European Wind Atlas, Part 1: Model Sensitivity, Geosci. Model Dev. Discuss., https://doi.org/10.5194/gmd-2019-349, in review, 2020.
Henckes, P., Knaut, A., Obermüller, F., and Frank, C.: The benefit of long-term high resolution wind data for electricity system analysis, Energy, 143, 934–942, https://doi.org/10.1016/j.energy.2017.10.049, 2018.
Heppelmann, T., Steiner, A., and Vogt, S.: Application of numerical weather prediction in wind power forecasting: Assessment of the diurnal cycle, Meteorol. Z., 26, 3, 319–331, https://doi.org/10.1127/metz/2017/0820, 2017.
Hersbach, H., Bell, B., Berrisford, P., Hirahara, S., Horányi, A., Muñoz-Sabater, J., Nicolas, J., Peubey, C., Radu, R., Schepers, D., Simmons, A., Soci, C., Abdalla, S., Abellan, X., Balsamo, G., Bechtold, P., Biavati, G., Bidlot, J., Bonavita, M., De Chiara, G., Dahlgren, P., Dee, D., Diamantakis, M., Dragani, R., Flemming, J., Forbes, R., Fuentes, M., Geer, A., Haimberger, L., Healy, S., Hogan, R. J., Hólm, E. A., Janisková, M., Keeley, S., Laloyaux, P., Lopez, P., Radnoti, G., Rosnay, P. D., Rozum, I., Vamborg, F., Villaume, S., and Thépaut, J.-N.: The ERA5 global reanalysis, Q. J. Roy. Meteorol. Soc., online first, https://doi.org/10.1002/qj.3803, 2020.
HErZ (Hans-Ertel Centre for Weather Research): COSMO-REA2 regional reanalysis, available at: https://reanalysis.meteo.uni-bonn.de/?Download_Data___COSMO-REA2, last access: 7 March 2020a.
HErZ (Hans-Ertel Centre for Weather Research): Post-processed radiation, available at: https://reanalysis.meteo.uni-bonn.de/?Derived_data_sets___Post-Processed_Radiation, last access: 3 July 2020b.
Hinrichs, I., Jahnke-Bornemann, A., Andersson, A., Ganske, A., Gouretski, V., Jensen, C., Klein, B., Möller, J., Sadikni, R., and Tinz, B.: The Baltic and North Seas Climatology (BNSC) – A Comprehensive, Observation-Based Data Product of Atmospheric and Hydrographic Parameters, Front. Earth Sci., 7, 158 pp., https://doi.org/10.3389/feart.2019.00158, 2019.
Jensen, T. V. and Pinson, P.: RE-Europe, a large-scale dataset for modeling a highly renewable European electricity system, Scientific Data, 4, 170175, https://doi.org/10.1038/sdata.2017.175, 2017.
Jourdier, B.: Evaluation of ERA5, MERRA-2, COSMO-REA6, NEWA and AROME to simulate wind power production over France, Adv. Sci. Res., 17, 63–77, https://doi.org/10.5194/asr-17-63-2020, 2020.
Kaiser-Weiss, A. K., Kaspar, F., Heene, V., Borsche, M., Tan, D. G. H., Poli, P., Obregon, A., and Gregow, H.: Comparison of regional and global reanalysis near-surface winds with station observations over Germany, Adv. Sci. Res., 12, 187–198, https://doi.org/10.5194/asr-12-187-2015, 2015.
Kaiser-Weiss, A. K., Borsche, M., Niermann, D., Kaspar, F. Lussana, C., Isotta, F., van den Besselaar, E., van der Schrier, G., and Undén, P.: Added value of regional reanalyses for climatological applications, Environ. Res. Commun., 1, 071004, https://doi.org/10.1088/2515-7620/ab2ec3, 2019.
Kaspar, F., Müller-Westermeier, G., Penda, E., Mächel, H., Zimmermann, K., Kaiser-Weiss, A., and Deutschländer, T.: Monitoring of climate change in Germany – data, products and services of Germany's National Climate Data Centre, Adv. Sci. Res., 10, 99–106, https://doi.org/10.5194/asr-10-99-2013, 2013.
Kaspar, F., Borsche, M., Pfeifroth, U., Trentmann, J., Drücke, J., and Becker, P.: A climatological assessment of balancing effects and shortfall risks of photovoltaics and wind energy in Germany and Europe, Adv. Sci. Res., 16, 119–128, https://doi.org/10.5194/asr-16-119-2019, 2019a.
Kaspar, F., Kratzenstein, F., and Kaiser-Weiss, A. K.: Interactive open access to climate observations from Germany, Adv. Sci. Res., 16, 75–83, https://doi.org/10.5194/asr-16-75-2019, 2019b.
Keller, J. D., Delle Monache, L., and Alessandrini, S.: Statistical Downscaling of a High-Resolution Precipitation Reanalysis Using the Analog Ensemble Method, J. Appl. Meteorol. Clim., 56, 2081–2095, https://doi.org/10.1175/JAMC-D-16-0380.1, 2017.
Kotlarski, S., Keuler, K., Christensen, O. B., Colette, A., Déqué, M., Gobiet, A., Goergen, K., Jacob, D., Lüthi, D., van Meijgaard, E., Nikulin, G., Schär, C., Teichmann, C., Vautard, R., Warrach-Sagi, K., and Wulfmeyer, V.: Regional climate modeling on European scales: a joint standard evaluation of the EURO-CORDEX RCM ensemble, Geosci. Model Dev., 7, 1297–1333, https://doi.org/10.5194/gmd-7-1297-2014, 2014.
Kreienkamp, F., Paxian, A., Früh, B., Lorenz, P., and Matulla, C: Evaluation of the empirical–statistical downscaling method EPISODES, Clim. Dynam., 52, 991–1026, https://doi.org/10.1007/s00382-018-4276-2, 2019.
Lockhoff, M., Zolina, O., Simmer, C., and Schulz, J.: Representation of Precipitation Characteristics and Extremes in Regional Reanalyses and Satellite- and Gauge-Based Estimates over Western and Central Europe, J. Hydrometeorol., 20, 1123–1145, https://doi.org/10.1175/JHM-D-18-0200.1, 2019.
Mesinger, F., DiMego, G., Kalnay, E., Mitchell, K., Shafran, P. C., Ebisuzaki, W., Jović, D., Woollen, J., Rogers, E., Berbery, E. H., Ek, M. B., Fan, Y., Grumbine, R., Higgins, W., Li, H., Lin, Y., Manikin, G., Parrish, D., and Shi, W.: North American Regional Reanalysis, B. Am. Meteorol. Soc., 87, 343–360, https://doi.org/10.1175/BAMS-87-3-343, 2006.
Naz, B. S., Kurtz, W., Montzka, C., Sharples, W., Goergen, K., Keune, J., Gao, H., Springer, A., Hendricks Franssen, H.-J., and Kollet, S.: Improving soil moisture and runoff simulations at 3 km over Europe using land surface data assimilation, Hydrol. Earth Syst. Sci., 23, 277–301, https://doi.org/10.5194/hess-23-277-2019, 2019.
Naz, B. S., Kollet, S., Franssen, H. H., Montzka, C., and Kurtz, W.: A 3 km spatially and temporally consistent European daily soil moisture reanalysis from 2000 to 2015. Sci Data, 7, 111, https://doi.org/10.1038/s41597-020-0450-6, 2020.
Niermann, D., Borsche, M., Kaiser-Weiss, A., van den Besselaar, E., van der Schrier, G., Cornes, R., de Vreede, E., Lussana, C., Tveito, O. E., Cantarello, L., Frei, C., Isotta, F., Davie, J., Bazil, E., and Bojariu, R.: UERRA Deliverable 3.6.: Scientific report on assessment of regional analysis against independent datasets, available at: http://www.uerra.eu/project-overview/all-deliverables.html (last access: 7 March 2020), 2018.
Niermann, D., Borsche, M., Kaiser-Weiss, A. K., and Kaspar, F.: Evaluating renewable energy relevant parameters of COSMO-REA6 by comparing against station observations, satellites and other reanalyses, Meteorol. Z., 28, 347–360, https://doi.org/10.1127/metz/2019/0945, 2019.
Ostermöller, J., Lorenz, P., Kreienkamp, F., Fröhlich, K., Martin-Santos, I., Holzmann, H., Herrnegger, M., and Simoes, S. G.: Generating highly resolved seasonal forecasts for hydropower and energy system modelling in the CLIM2POWER project. European Meteorological Society Annual Meeting Abstracts, Vol. 16, EMS2019-251, available at: https://meetingorganizer.copernicus.org/EMS2019/EMS2019-251.pdf (last access: 3 July 2020), 2019.
Paeth, H., Pollinger, F., Mächel, H., Figura, C., Wahl, S., Ohlwein, C. and Hense, A.: An efficient model approach for very high resolution orographic precipitation, Q. J. R. Meteor. Soc., 143, 2221–2234, https://doi.org/10.1002/qj.3080, 2017.
Peter, J. and Wagner, J.: Optimal allocation of variable renewable energy considering contributions to security of supply, EWI Working Papers, Energiewirtschaftliches Institut an der Universität zu Köln (EWI), 2018.
Pickering, B., Grams, C. M., and Pfenninger, S.: Sub-national variability of wind power generation in complex terrain and its correlation with large-scale meteorology, Environ. Res. Lett., 15, 04402, https://doi.org/10.1088/1748-9326/ab70bd, 2020.
Potthast, R., Walter, A., and Rhodin, A.: A Localised Adaptive Particle Filter within an Operational NWP Framework, Mon. Weather Rev., 147, 345–362, https://doi.org/10.1175/MWR-D-18-0028.1, 2019.
PV-GIS: Preliminary description of the new reanalysis-based data in PVGIS, Joint Research Center, https://ec.europa.eu/jrc/en/PVGIS/docs/reanalysis, last access: 1 March 2020.
Ramirez Camargo, L., Nitsch, F., Gruber, K., and Dorner, W.: Electricity self-sufficiency of single-family houses in Germany and the Czech Republic, Appl. Energ., 228, 902–915, https://doi.org/10.1016/j.apenergy.2018.06.118, 2018.
Ramirez Camargo, L., Gruber, K., and Nitsch, F.: Assessing variables of regional reanalysis data sets relevant for modelling small-scale renewable energy systems, Renew. Energ., 133, 1468–1478, https://doi.org/10.1016/j.renene.2018.09.015, 2019a.
Ramirez Camargo, L., Nitsch, F., Gruber, K., Valdes, J., Wuth, J., and Dorner, W.: Potential Analysis of Hybrid Renewable Energy Systems for Self-Sufficient Residential Use in Germany and the Czech Republic, Energies, 12, 4185, https://doi.org/10.3390/en12214185, 2019b.
Rasquin, C., Seiffert, R., Wachler, B., and Winkel, N.: The significance of coastal bathymetry representation for modelling the tidal response to mean sea level rise in the German Bight, Ocean Sci., 16, 31–44, https://doi.org/10.5194/os-16-31-2020, 2020.
Scherrer, S. C.: Temperature monitoring in mountain regions using reanalyses: lessons from the Alps. Environ. Res. Lett., 15, 044005, https://doi.org/10.1088/1748-9326/ab702d, 2020.
Schraff, C. and Hess, R.: A description of the non-hydrostatic regional model LM – Part III: Data assimilation, Technical report, Deutscher Wetterdienst, Offenbach, Germany, 2003.
Schraff, C., Reich, H., Rhodin. A., Schomburg, A., Stephan, K., Perianez, A., and Potthast, R.: Kilometer Scale Ensemble Data Assimilation for the COSMO Model (KENDA), Q. J. Roy. Meteor. Soc., 142, 696, 1453–1472, https://doi.org/10.1002/qj.2748, 2016.
Schättler, U., Doms, G., and Schraff, C.: A description of the non-hydrostatic regional model LM – Part VII: Users' guide, Technical report, Deutscher Wetterdienst, Offenbach, Germany, 2011.
Schimanke, S., Undén, P., Isaksson, L., Edvinsson, L., Ridal, M., Olsson, E., Hopsch, S., and Andersson, S.: Copernicus regional reanalysis for Europe, European Meteorological Society Annual Meeting Abstracts, 16, EMS2019-134, https://meetingorganizer.copernicus.org/EMS2019/EMS2019-134.pdf (last access: 3 July 2020), 2019.
Simmer, C., Adrian, G., Jones, S., Wirth, V., Göber, M., Hohenegger, C., Janjic, T., Keller, J., Ohlwein, C., Seifert, A., Trömel, S., Ulbrich, T., Wapler, K., Weissmann, M., Keller, J., Masbou, M., Meilinger, S., Riß, N., Schomburg, A., Vormann, A., and Weingärtner, C.: HErZ: The German Hans-Ertel Centre for Weather Research, B. Am. Meteorol. Soc., 97, 1057–1068, https://doi.org/10.1175/BAMS-D-13-00227.1, 2016.
Springer, A., Eicker, A., Bettge, A., Kusche, J., and Hense, A.: Evaluation of the Water Cycle in the European COSMO-REA6 Reanalysis Using GRACE, Water, 9, 289, https://doi.org/10.3390/w9040289, 2017.
Steinheuer, J. and Friederichs, P.: Vertical profiles of wind gust statistics from a regional reanalysis using multivariate extreme value theory, Nonlin. Processes Geophys., 27, 239–252, https://doi.org/10.5194/npg-27-239-2020, 2020.
Steinke, S., Wahl, S., and Crewell, S.: Benefit of high resolution COSMO reanalysis: The diurnal cycle of column-integrated water vapor over Germany. Meteorol. Z., 28, 165–177, https://doi.org/10.1127/metz/2019/0936, 2019.
Stöckli, R.: The HelioMont Surface Solar Radiation Processing (2017 Update). Scientific Report Meteo Swiss 93, 126 pp., 2003.
Su, C.-H., Eizenberg, N., Steinle, P., Jakob, D., Fox-Hughes, P., White, C. J., Rennie, S., Franklin, C., Dharssi, I., and Zhu, H.: BARRA v1.0: the Bureau of Meteorology Atmospheric high-resolution Regional Reanalysis for Australia, Geosci. Model Dev., 12, 2049–2068, https://doi.org/10.5194/gmd-12-2049-2019, 2019.
Tinz, B., Outzen, O., Kaspar, F., and Gehrke, M.: Meteorologische und ozeanographische Daten für den weiteren Ausbau der Windenergie in der Ausschließlichen Wirtschaftszone Deutschlands. Kurzfassungen der Meteorologentagung DACH, Garmisch-Partenkirchen, Deutschland, 18–22 März 2019, DACH2019-338, available at: https://www.dach2019.de/DACH2019-abstracts.pdf (last access: 3 July 2020), 2019.
Urraca, R., Huld, T., Gracia-Amillo, A., Martinez-de-Pison, F. J., Kaspar, F., and Sanz-Garcia, A.: Evaluation of global horizontal irradiance estimates from ERA5 and COSMO-REA6 reanalysis using ground and satellite-based data, Sol. Energy, 164, 339–354, https://doi.org/10.1016/j.solener.2018.02.059, 2018a.
Urraca, R., Huld, T., Javier Martinez-de-Pison, F., and Sanz-Garcia, A.: Sources of uncertainty in annual global horizontal irradiance data, Sol. Energy, 170, 873–884, https://doi.org/10.1016/j.solener.2018.06.005, 2018b.
Wachler, B., Rasquin, C., Seiffert, R., and Kösters, F.: Tidal response to sea level rise and bathymetric changes in the German Wadden Sea, Ocean Dynam., https://doi.org/10.1007/s10236-020-01383-3, 2020.
Wahl, S., Bollmeyer, C., Crewell, S., Figura, C., Friederichs, P., Hense, A., Keller, J. D., and Ohlwein, C.: A novel convective-scale regional reanalyses COSMO-REA2: Improving the representation of precipitation, Meteorol. Z., 26, 345–361, https://doi.org/10.1127/metz/2017/0824, 2017.
Wiesner, S., Ament, F., Beyrich, F., Duran, I., Klocke, D., Hohenegger, C., Löhnert, U., Göber, M., Masbou, M., Rust, H., Schlemmer, L., Schmidli, J., Schomburg, A., Wahl, S., and Weissmann, M.: FESSTVaL: Field Experiment on sub-mesoscale spatio-temporal variability in Lindenberg – the campaign is at the ready. European Meteorological Society Annual Meeting Abstracts, Vol. 16, EMS2019-607-1, available at: https://meetingorganizer.copernicus.org/EMS2019/EMS2019-607-1.pdf (last access: 3 July 2020), 2019.
WIK: mFUND Projekte im Porträt – 7 Fragen an KISP. Eine Veröffentlichung der mFUND-Begleitforschung des Wissenschaftlichen Instituts für Infrastruktur und Kommunikationsdienste, available at: https://www.bmvi.de/SharedDocs/DE/Anlage/DG/mFUND/mfund-kisp.pdf?__blob=publicationFile (last access: 3 July 2020), 2019.
Zängl, G., Reinert, D., Rípodas, P., and Baldauf, M.: The ICON (ICOsahedral Non-hydrostatic) modelling framework of DWD and MPI-M: Description of the non-hydrostatic dynamical core, Q. J. Roy. Meteor. Soc., 141, 563–579, https://doi.org/10.1002/qj.2378, 2015.