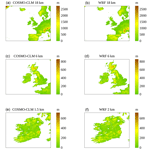
Towards a definitive historical high-resolution climate dataset for Ireland – promoting climate research in Ireland
Jason Flanagan
Paul Nolan
Ray McGrath
Christopher Werner
There is strong and constant demand from various sectors (research, industry and government) for long-term, high-resolution (both temporal and spatial), gridded climate datasets. To address this demand, the Irish Centre for High-End Computing (ICHEC) has recently performed two high-resolution simulations of the Irish climate, utilising the Regional Climate Models (RCMs) COSMO-CLM5 and WRF v3.7.1. The datasets produced contain hourly outputs for an array of sub-surface, surface and atmospheric fields for the entire 36-year period 1981–2016. In this work, we list the climate variables that have been archived at ICHEC. We present preliminary uncertainty estimates (error, standard deviation, mean absolute error) based on Met Éireann station observations, for several of the more commonly used variables: 2 m temperature, 10 m wind speeds and mean sea level pressure at the hourly time scale; and precipitation at hourly and daily time scales. Additionally, analyses of 10 cm soil temperatures, CAPE 3 km, Showalter index and surface lifted index are presented.
- Article
(7676 KB) - Full-text XML
- BibTeX
- EndNote
Gridded climate datasets are invaluable aids to studies in observed climate change trends and variability. Additionally, they have potential application to many other diverse areas of interest – agriculture, hydrology, renewable energy (wind, wave and solar), public health and socio-economic planning. In Ireland, station observations have traditionally been used to describe the Irish climate (in conjunction with satellite observations) and produce gridded datasets. For instance, daily and monthly gridded datasets (at 1 km resolution) of precipitation have been created for Ireland (Walsh, 2012, 2016) and are based on station data from Met Éireann's rainfall network – the identification of changes in Irish precipitation patterns, whether they be driven by natural variability or man-made climate change, is particularly important to the country with recent projections pointing to an increased likelihood of summer droughts and winter flooding (Nolan et al., 2013a, b). Unfortunately, gridded datasets based on station observations come with numerous caveats as detailed by Prein and Gobiet (2017): they may not be particularly representative in regions with few stations; station data are prone to error and/or missing values; precipitation under-catch and excessive smoothing.
The outputs from numerical weather models represent an alternative to observations for the production of gridded datasets. The European Centre for Medium-Range Weather Forecast (ECMWF) has initiated several global reanalysis datasets beginning with ERA-15 (1979–1993; 190 km resolution; Gibson et al., 1997). As the models used have improved, finer resolution datasets have followed: ERA-40 (1957–2002; 125 km; Uppala et al., 2005); ERA-Interim (1979–present; 80 km; Dee et al., 2011) and more recently ERA5 (1950–present; 31 km).
Regional reanalysis and dynamical downscaling are two methods often used to achieve higher resolution (and overcome the associated computational cost). Both methods make use of forcing by global reanalysis data at the boundaries, with the former assimilating regional data and the latter making use of nested domains (without data assimilation). In recent years, numerous regional reanalyses and downscaled products have been published – two examples of the former being HIRLAM (1979–2014; 22 km; Dahlgreen et al., 2016) and COSMO-REA6 (1997–2004; 6 km; Bollmeyer et al., 2015) whilst two examples of the latter are described in Lucas-Picher et al. (2012) and Dasari and Challa (2015). There are both advantages and disadvantages to the downscaling approach: downscaling can offer both finer detail and less computational cost than regional reanalysis (Kanamitsu and Kanamaru, 2007); errors are cascaded with new errors introduced through the flow of information at the boundaries. There are however, many examples that illustrate the method's ability to simulate precipitation (Kendon et al., 2012; Lucas-Picher et al., 2012), near-surface temperature (Di Luca et al., 2016) and winds (Feser et al., 2011) at high resolution.
Although there are numerous high-resolution regional reanalysis datasets available, up until recently (2017), there have been none that cover Ireland at spatial resolutions higher than 6 km. In 2017, Met Éireann completed a 36-year simulation (MÉRA) at 2.5 km resolution for the period 1981–2016 (Gleeson et al., 2017). The MÉRA datasets, which are stored as a series of 3 and 33 h forecasts, have been archived by Met Éireann at 1 h intervals. A full description of the available data and some associated preliminary uncertainty estimates are given in Whelan et al. (2017) and Gleeson et al. (2017).
Additionally, in 2017, two high-resolution simulations of the Irish Climate, covering the period 1981–2016, were completed by the Irish Centre for High-End Computing (ICHEC). The simulations were achieved by downscaling ERA-Interim data using the RCMs; the Weather Research and Forecasting model (WRF v3.7.1) (Skamarock et al., 2008) and COSMO-CLM5 (Rockel et al., 2008). The RCMs were run at 2 and 1.5 km spatial resolution respectively, with two additional 6 and 18 km simulations for both models. The data produced by each ICHEC reanalysis has been archived at 1 h intervals. Although the MÉRA resolution is lower than those of the two ICHEC simulations, it does have the advantage of data assimilation (optimal interpolation for surface parameters, 3DVAR assimilation for upper-air parameters). Together, the three datasets constitute a first step toward the production of definitive, high-resolution, gridded climate datasets for Ireland.
In Sect. 2, a description of the model setup for each of the ICHEC simulations is given and a description of the climate variables archived at ICHEC is provided. In Sect. 3, uncertainty estimates (error, standard deviation and mean absolute error) utilising station observations are assigned to some of the basic parameters (precipitation, 2 m temperature, 10 m wind speeds and mean sea-level pressure). Finally, in Sect. 4, the results and plans for future work are discussed.
2.1 Model setups
Both the ICHEC WRF and COSMO-CLM RCM simulations were performed utilising nested domains with 18, 6, and 2 km (WRF) or 1.5 km (COSMO-CLM) resolutions. Figure 1 illustrates the spatial coverage and topography of the three COSMO-CLM and WRF domains. The WRF 18 km domain is composed of a 176×183 grid with latitudinal extent 36.76 to 67.56∘ N and longitudinal extent 42.15∘ W to 24.15∘ E, the 6 km domain is on a 216×210 grid with latitudinal extent 47.74 to 59.52∘ N and longitudinal extent 17.78∘ W to 4.72∘ E and the 2 km domain is on a 216×273 grid with latitudinal extent 50.81 to 55.77∘ N and longitudinal extent 11.57 to 4.71∘ W. The three COSMO-CLM domains are only slightly larger than the WRF domains: 18 km on a 188×188 grid with latitudinal extent 35.51 to 68.36∘ N and longitudinal extent 45.54∘ W to 27.26∘ E; 6 km on a 245×245 grid with latitudinal extent 46.84 to 60.45∘ N and longitudinal extent 20.16∘ W to 5.76∘ E; and 1.5 km on a 328×398 grid with latitudinal extent 50.64 to 56.04∘ N and longitudinal extent 11.93 to 4.11∘ W. The 18 km simulations were driven at the boundaries by ERA-Interim reanalysis data, produced by ECMWF at 80 km resolution, with all outputs (Sect. 2.2) from each individual nested domain (for both COSMO-CLM and WRF) archived at hourly intervals.
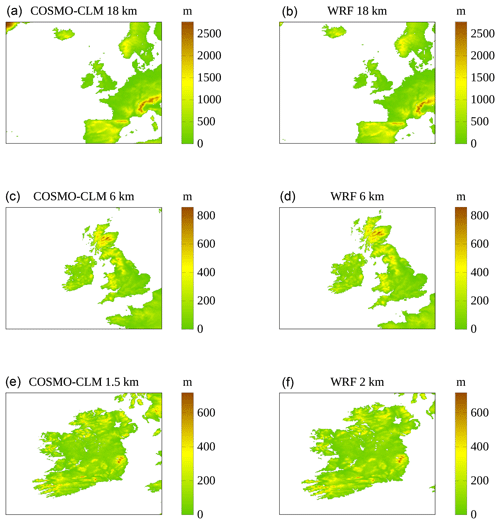
Figure 1The nested domains used for the COSMO-CLM (a, c, e) and WRF (b, d, f) model runs showing model topography at three spatial resolutions; 18 km (a, b), 6 km (c, d) and 2 km (e, f).
The WRF model used here (v3.7.1) comes with topography data at four resolutions (10, 5, 2 and 0.5 arcmin) that can be used to construct terrain data for the model grid. Given that some climate variables (e.g. winds) are affected by nearby topography, it was realised that underlying data with much finer resolution was required. Therefore, a 1 arcsec topography dataset (The Advanced Spaceborne Thermal Emission and Reflection Radiometer (ASTER) Global Digital Elevation Model (GDEM)) was obtained and incorporated into the WRF simulations using the WRF Preprocessing System (WPS). By contrast, the COSMO-CLM model already includes the high-resolution ASTER topography dataset as part of the preprocessing stage (ExtPAR).
Both models have numerous parameter schemes that can potentially affect outputs. For instance, it is known that the choice of WRF sub-grid orographic, flow blocking and gravity wave drag schemes can influence bias in 10 m wind speeds, 2 m temperature and surface pressure (Koo et al., 2018). To ensure the most accurate options were employed, the results from several 1-month validation simulations previously performed at ICHEC (Nolan et al., 2017) were utilised. Summaries of the individual model settings, where different from the default option (or between different resolutions) are given in Tables 1 and 2.
2.2 Model outputs
All outputs from each of the 18 km, 6 km and highest resolution 2 km (WRF) and 1.5 km (COSMO-CLM) RCM simulations have been archived at hourly intervals by ICHEC. A full listing of all climate parameters (and their relevant units) archived at ICHEC is given in Tables 3–5 (COSMO-CLM) and 6–8 (WRF). The COSMO-CLM dataset is composed of 48 surface/near-surface parameters (Table 3), two sub-surface parameters at eight levels (Table 4) and three upper-air parameters at 10 heights (Table 5). The WRF dataset contains 23 surface/near-surface parameters (Table 6), two sub-surface parameters at four levels (Table 7) and two upper-air parameters at five levels (Table 8).
Table 3COSMO-CLM surface or near-surface parameters (with units) archived by ICHEC at 1 h intervals.
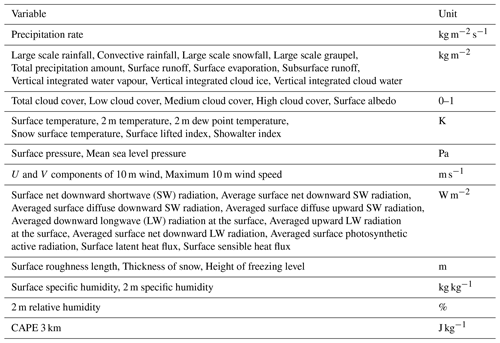
Table 4COSMO-CLM sub-surface parameters (with units) at 8 levels (0.005, 0.02, 0.06, 0.18, 0.54, 1.62, 4.86 and 14.58 m) archived by ICHEC at 1 h intervals.
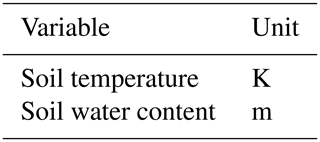
Table 5COSMO-CLM upper-air parameters (with units) at 10 heights (20, 40, …, 200 m) archived by ICHEC at 1 h intervals.
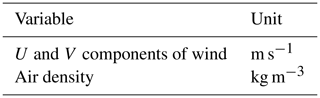
Table 7WRF sub-surface parameters (with units) at 4 levels (5, 25, 75 and 150 cm below the surface) archived by ICHEC at 1 h intervals.
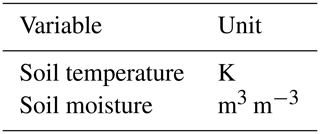
Table 8WRF upper-air parameters (with units) at 5 heights (40, 60, 80, 100, 120 m) archived by ICHEC at 1 h intervals.
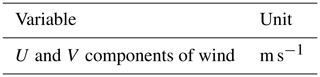
The climate parameters presented here constitute the highest (spatial) resolution, hourly dataset currently available for Ireland for the period 1981–2016 (data for 2017 is nearing completion and will soon be added to the dataset). Additionally, the datasets contain parameters that are currently not available elsewhere (MÉRA or observations). These new high-resolution parameters have potential for many applications and will be of use to researchers from different fields. For instance: hydrology (surface and subsurface runoff); wind energy (air density at turbine heights); extreme events (CAPE 3 km, Showalter index, surface lifted index); and agriculture (soil temperature and moisture content at four (WRF) and eight (COSMO-CLM) levels).
Basic uncertainty estimates (error, standard deviation and mean absolute error) have been calculated for the more commonly-used parameters: 2 m temperature, 10 m wind speed, pressure and precipitation. All estimates are at the hourly time scale, with the inclusion of mean daily error for precipitation, and are based on observations from Met Éireann's 25 synoptic stations (hourly) and 484 station rainfall network (daily/monthly). The results of these analyses are presented in Sect. 3.1–3.4. Additionally, preliminary analyses of less commonly-used parameters (10 cm soil temperature, CAPE 3 km, Showalter and surface lifted indices) have been performed utilising station and/or radiosonde data at appropriate timescales and are described in Sect. 3.5 and 3.6.
Gridded datasets of observed daily (00:00–00:00 UTC) temperature and (09:00–09:00 UTC) accumulated precipitation, at 1 km resolution, covering the Republic of Ireland for the period 1981–2015 have been obtained from Met Éireann and form part of a preliminary qualitative comparison detailed below. Comprehensive details concerning the production of these datasets are provided in Walsh (2017) (temperature) and Walsh (2016) (precipitation).
Additionally, hourly MÉRA precipitation and 2 m temperature data has been obtained for the same period. The MÉRA data is contained within three-hour and 33 h forecast files (each containing hourly forecasts) and have been converted to daily records in the following ways: for precipitation, records have been built utilising 33 h (accumulated precipitation) forecast files (thereby avoiding any negative impact from spin-up through use of the 3 h forecast files) and consecutive subtractions of the 9 h forecast from the 33 h forecast for each day; for 2 m temperature, hourly values were first obtained from the 3 h files, followed by daily averaging. Daily records are relatively straightforward to derive from the COSMO-CLM and WRF temperature and precipitation datasets – each daily record is simply the mean of hourly values over the relevant 00:00–00:00 UTC period for temperature, and the sum of hourly values over the relevant 09:00–09:00 UTC period for precipitation. Annual records for the gridded observations and each of the three models are then easily obtained through summation (precipitation) and averaging (2 m temperature).
The average annual 2 m temperature and precipitation amounts, derived from each of the available gridded datasets are shown in Figs. 2 and 3, respectively. For temperature, all four datasets show similar spatial distribution – particularly (to-be-expected) cooler temperatures in the north and over mountains. However, there is a distinct warm region visible over the midlands that diminishes in strength as we move from the observations-based dataset and on to the COSMO-CLM, MÉRA and WRF datasets, in turn. For precipitation, all four datasets again show similar spatial distribution with higher rainfall amounts in the west and over mountains, with particularly strong agreement between the observations-based and WRF datasets in these regions. Additionally, each of the three model datasets show greater agreement in eastern regions – there is a drier trend in the observations dataset that is not as evident in the COSMO-CLM, WRF and MÉRA datasets.
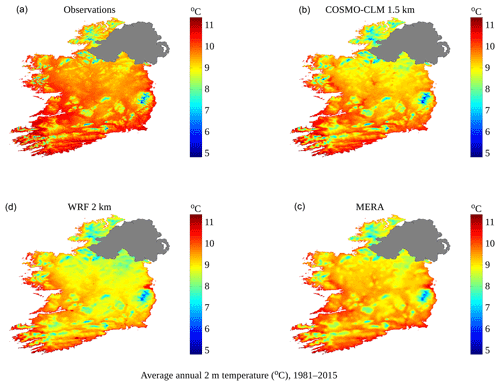
Figure 2Average annual temperature (∘C) for the period 1981–2015. Shown are the values found from (working clockwise): (a) gridded observations provided by Met Éireann; (b) the COSMO-CLM 1.5 km dataset; (c) the MÉRA dataset; (d) the WRF 2 km dataset.
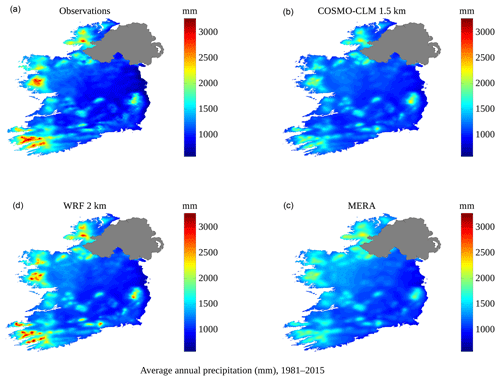
Figure 3Average annual rainfall (mm) over the period 1981–2015. Shown are the values found from (working clockwise): (a) gridded observations provided by Met Éireann; (b) the COSMO-CLM 1.5 km dataset; (c) the MÉRA dataset; (d) the WRF 2 km dataset.
3.1 Hourly 2 m temperature
Hourly station observations of air temperature have been obtained from Met Éireann. In total, there are 25 of these stations with varying record lengths available. The COSMO-CLM and WRF datasets have been processed so that a comparison with these observations could be made – the 2 m temperature data is already stored in hourly files and data at the relevant station locations can be extracted immediately through (bilinear) interpolation. Overall values have been determined by treating all available station data as a single dataset. The (mean) error values found are −0.18 ∘C (COSMO-CLM) and −0.31 ∘C (WRF), whilst the overall standard deviations are 1.79 ∘C (COSMO-CLM) and 1.71 ∘C (WRF). Additionally, the mean absolute error (MAE) has been calculated (using the entire station dataset) – the values found are 1.34 ∘C (COSMO-CLM) and 1.31 ∘C (WRF). For comparison, an identical analysis of hourly MÉRA 2 m temperature yields the values −0.10 ∘C (mean error), 1.09 ∘ (standard deviation) and 0.81 ∘C (MAE).
In Fig. 4, the 2 m temperature error distributions for COSMO-CLM and WRF are shown to provide insight into the performance of each model. Overall, both COSMO-CLM and WRF display somewhat similar error, with WRF slightly more likely to be within 1 ∘C of observed values. Except for the error range , WRF is consistently more likely than COSMO-CLM to underestimate 2 m temperature. In turn, COSMO-CLM shows a higher frequency of large positive error. Additionally, whilst the mean errors and standard deviations of both models are similar for the left-most error range (−4.83 and 0.82 ∘C (COSMO-CLM) and −4.85 and 0.81 ∘C (WRF) respectively) COSMO-CLM produces larger positive outliers (mean error =5.38 ∘C and standard deviation =1.33 ∘C versus 5.10 and 1.10 ∘C respectively, for WRF).
3.2 Hourly 10 m wind speeds
Hourly 10 m wind speed synoptic station observations have also been obtained from Met Éireann. For both WRF and COSMO-CLM, the 10 m U and V wind components have been found at each station location through bilinear interpolation and used to calculate 10 m wind speeds () which are then compared to observations. Overall mean error values found are 0.85 m s−1 (COSMO-CLM) and 0.07 m s−1 (WRF), whilst the overall standard deviations are 2.30 m s−1 (COSMO-CLM) and 2.24 m s−1 (WRF). The overall MAEs found are 1.89 m s−1 (COSMO-CLM) and 1.67 m s−1 (WRF). For comparison, an identical analysis of hourly MÉRA 10 m wind speeds yields the values 0.29 m s−1 (mean error), 1.65 m s−1 (standard deviation) and 1.27 m s−1.
Figure 5 shows the 10 m wind speed error distributions found for COSMO-CLM and WRF. It can be readily seen that the COSMO-CLM distribution exhibits greater frequency of error at higher ranges. An opposite, but weaker, behaviour is evident for negative ranges where WRF shows higher frequencies. At the extremes (less than −4 m s−1 and greater than 4 m s−1) both COSMO-CLM and WRF exhibit similar mean errors (approximately −5.5 and 5.3 m s−1, respectively) and standard deviations (1.5 and 1.3 m s−1, respectively).
3.3 Hourly sea-level pressure
A similar analysis to that in Sect. 3.1 and 3.2 has been performed for sea-level pressures utilising synoptic station data from Met Éireann. The overall (mean) error values found are −0.87 hPa (COSMO-CLM) and −0.20 hPa (WRF), whilst the overall standard deviations are 2.56 hPa (COSMO-CLM) and 2.39 hPa (WRF). The overall MAEs found are 1.96 hPa (COSMO-CLM) and 1.69 hPa (WRF). By comparison, an identical analysis of hourly MÉRA sea-level pressures gives the values 0.03 hPa (mean error), 0.51 hPa (standard deviation) and 0.37 hPa (MAE).
The COSMO-CLM and WRF error distributions are shown in Fig. 6, where COSMO-CLM has greater frequency at all negative ranges, whilst an opposite but weaker effect occurs at positive ranges. At extreme ranges (less than −4 hPa and greater than 4 hPa) both models exhibit similar mean errors (−6 and 6 hPa, respectively) and standard deviations (2.2 and 2.1 hPa, respectively).
3.4 Precipitation
An analysis of hourly precipitation amounts has been performed, again using synoptic station data sourced from Met Éireann. Both COSMO-CLM and WRF show remarkably similar error and standard deviations – overall error values are less than 0.01 mm and overall standard deviations are 0.63 mm, for both models. Additionally, the MAEs found are 0.18 mm for both models. By comparison, an identical analysis performed for hourly MÉRA precipitation results in the values: <0.01 mm (error), 0.55 mm (standard deviation) and 0.16 mm (MAE).
Figure 7 presents the error distributions for both models for four different observed precipitation categories: dry (0 mm), light (up to 0.2 mm), moderate (0.2–2.5 mm) and heavy (>2.5 mm).
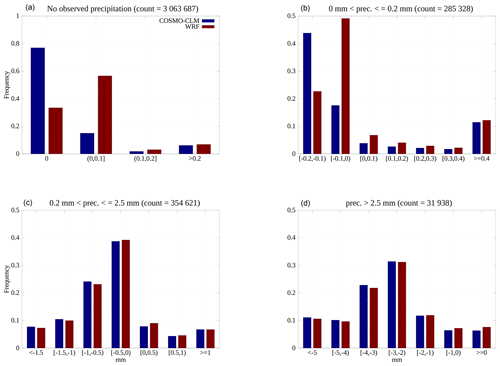
Figure 7Bar graphs showing the precipitation error distributions found for the COSMO-CLM (in blue) and WRF (in red) models and categorised according to observed hourly station amounts. (a) No precipitation; (b) light precipitation (0 mm, 0.2 mm]; (c) moderate precipitation (0.2 mm, 2.5 mm]; (d) heavy precipitation, >2.5 mm.
For no observed precipitation (Fig. 7, top-left panel), the count (3 063 887) is approximately 4.6 times higher than all other categories combined (671 887) – it does not rain as often as is commonly perceived. In this category, COSMO-CLM has much higher frequency of correct predictions. However, the overall error and standard deviation of both models are similar (0.07 and 0.4 mm, respectively). This is a result of COSMO-CLM producing larger (although fewer) errors than WRF – the means for the highest error range (>0.2 mm) are 1 and 0.9 mm, respectively.
For light rainfall (Fig. 7, top-right panel), both models display higher frequency of negative error, with WRF exhibiting higher frequency of low error. However, both show similar frequencies, means (1.3 mm) and standard deviations (1.3 mm) at the extreme error range (≥0.4 mm) which contribute to similar overall errors (>0.1 mm) and standard deviations (0.65 mm) for this category.
Both models exhibit similar error distributions for the moderate (Fig. 7, bottom-left panel) and heavy (Fig. 7, bottom-right panel) precipitation categories. For moderate precipitation, both models under-predict with the majority (∼0.85) of error values falling in the combined range [−1 mm, 0 mm). The overall errors and standard deviations for this category are similar: −0.38 and 1.05 mm (COSMO-CLM) and −0.35 and 1.01 mm (WRF). The frequencies of large negative and positive error are both less than 0.1. Both models exhibit similar mean errors and standard deviations for the error range <1.5 mm: −1.9 and 0.27 mm respectively. For the error range ≥1 mm, the corresponding values are slightly different: 2.35 and 1.8 mm (COSMO-CLM) and 2.23 and 1.6 mm (WRF).
Heavy precipitation is relatively rare in Ireland – the overall observed count (31 938) is an order of magnitude lower than that of the other two wet categories. For this category, both models typically under-predict, with WRF performing only marginally better than COSMO-CLM – the overall mean errors and standard deviations are −2.95 and 2.33 mm for COSMO-CLM and −2.84 and 2.32 mm for WRF. Indeed, the frequency of error in the range ≥0 mm is less than 0.1 for both models, with WRF performing slightly better – mean errors and standard deviations are 1.88 and 2.37 mm for COSMO-CLM and 1.63 and 1.98 mm for WRF. The frequencies, mean error (−7 mm) and standard deviation (2.5 mm) of large negative error are similar for both models, with WRF exhibiting only slightly lower frequency than COSMO-CLM.
Daily precipitation amounts have been obtained from Met Éireann's 484 station rainfall network and used to estimate mean 24 h accumulation errors for both COSMO-CLM (Fig. 8) and WRF (Fig. 9). Overall mean errors, standard deviations and MAEs found for COSMO-CLM are −0.23, 5.94 and 2.97 mm, respectively, whilst for WRF the respective values found are 0.10, 5.41 and 2.69 mm. By comparison, an identical analysis of MÉRA produces the values −0.09, 4.59 and 2.28 mm. For both COSMO-CLM and WRF, the largest errors occur over regions with complex topography (mountainous regions in the west) and during autumn and winter months when rainfall amounts tend to be largest. Both models tend to under-predict during drier spring and summer months – the mean errors found for WRF are −0.45 and −0.26 mm respectively, whilst for COSMO-CLM, the values found are −0.002 and −0.01 mm. Although COSMO-CLM shows better accuracy than WRF during drier seasons, the reverse is true during autumn and winter, when rainfall amounts are higher and both models over-predict – the mean values found for WRF are 0.29 and 1.20 mm respectively, whilst for COSMO-CLM the values found are 0.43 and 1.45 mm.
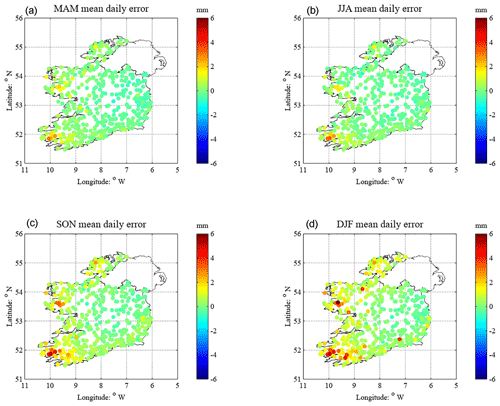
Figure 8COSMO-CLM daily precipitation error (by season) for the period 1981–2015, found utilising Met Éireann daily station data for: (a) Spring (MAM); (b) Summer (JJA); (c) Autumn (SON); (d) Winter (DJF).
3.5 10 cm soil temperature
An analysis of daily mean 10 cm soil temperatures has been performed through comparison with daily values from 23 Met Éireann stations. Simple linear vertical interpolation (cdo command intlevel, Schulzweida, 2018) has been used to generate model data at this level from the archived levels (Tables 4 and 7). Horizontal bilinear interpolation to the station locations has then been applied for all stations surrounded by land points. Where a station is next to a sea point, a simple nearest-neighbour approach was taken. For those stations where bilinear interpolation was possible, the nearest-neighbour method was tested and compared – absolute differences in mean temperature values were small (typically less than 0.1 ∘C) and usually in favour of the bilinear method. The overall daily errors, standard deviations and MAEs found were 1.17, 1.26 and 1.37 ∘C (COSMO-CLM) and 1.01, 1.16 and 1.18 ∘C (WRF).
The mean daily errors per season for each station are shown in Figs. 10 (COSMO-CLM) and 11 (WRF). Both models exhibit positive mean error for each season (MAM, JJA, SON, DJF) with least error during colder seasons: (1.34, 1.79, 0.76, 0.78 ∘C) COSMO-CLM; (1.08, 1.62, 0.67, 0.68 ∘C) WRF. By comparison, Gleeson et al. (2017) (their Fig. 8a) show lower mean error over the equivalent time periods, albeit for 20 cm soil temperature, with consistent over- (under-) prediction during winter (summer) months.
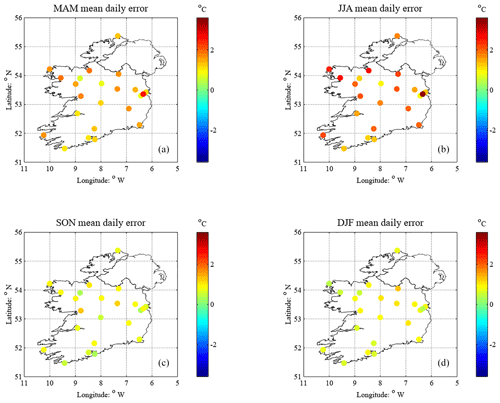
Figure 10COSMO-CLM mean daily 10 cm soil temperature error per season found through comparison with observations from 23 Met Éireann station data for: (a) Spring (MAM); (b) Summer (JJA); (c) Autumn (SON); (d) Winter (DJF).
The standard deviations found were (1.47, 1.92, 1.08, 1.01 ∘C) for COSMO-CLM and (1.25, 1.72, 0.85, 0.88 ∘C) for WRF whilst the MAEs found were (1.19, 1.35, 1.13, 1.07 ∘C) for COSMO-CLM and (1.20, 1.33, 0.87, 0.93 ∘C) for WRF. The two models show similar MAEs during spring and summer months, whereas WRF shows greater accuracy during autumn and winter months when temperatures are lower. From Figs. 10 and 11, there does not appear to be a pattern to the spatial distribution of errors. However, this could simply be due to the lack of observational data available – 23 stations here compared to 484 for the rainfall analysis in Sect. 3.4.
3.6 CAPE 3 km, Showalter and surface lifted indices
A preliminary analysis of COSMO-CLM parameters that are potentially of interest to researchers of weather extremes has been conducted. Observational data for these parameters are both rare and difficult to obtain. However, radiosonde data has been obtained for two locations in Ireland: Valentia and Castor Bay. The Valentia data covers the period 1981–present, whilst the Castor Bay data covers the period 2003–present. Typically, the soundings are recorded every 6 hours beginning at midnight on each day.
For CAPE 3 km, the overall errors, standard deviations and MAEs found for Valentia are 2.31, 47.5 and 2.31 J kg−1, respectively. For Castor Bay, the equivalent values are −0.68, 34.9 and 10.33 J kg−1. The Showalter index results at Valentia are −0.05, 3.51 and 2.58 whilst at Castor Bay the values are quite similar: 0.43, 3.36 and 2.52. The surface lifted index results are 0.06, 3.03 and 2.27 (Valentia) and 1.08, 3.09 and 2.58 (Castor Bay).
The error distributions for each parameter at Valentia and Castor Bay are shown in Figs. 12 and 13, respectively. For each parameter and location, the distributions typically have long tails – evidenced by the relatively large frequencies at the extremes of each as well as the means and standard deviations found for these ranges. For CAPE 3 km at Valentia, the respective values are −64.5 and 105.3 J kg−1 ( range) and 55.3 and 36.6 J kg−1 (>15 range). At Castor Bay, the equivalent values found are −55.5 and 88.9 J kg−1, and 49.0 and 35.0 J kg−1 respectively. For Showalter index at Valentia, the respective values are −7.7 and 2.4 ( range) and 6.7 and 1.9 (≥5 range). At Castor Bay, the equivalent values are similar: −7.7 and 2.5, and 6.6 and 1.6 respectively. Finally, for Valentia surface lifted index, the respective values are −7.2 and 2.2 ( range) and 6.5 and 1.8 (≥5 range), whilst at Castor Bay, the equivalent values are (again) similar: −7.1 and 2.1, and 6.4 and 1.4 respectively.
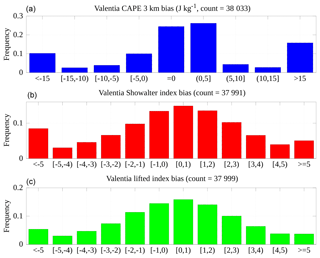
Figure 12Error distributions for three COSMO-CLM parameters, found through comparison with Valentia radiosonde data. (a) Cape 3 km. (b) Showalter index. (c) surface lifted index.
We have described the model setups and the (hourly) climate parameters output from two high-resolution downscaled simulations (using the RCMs COSMO-CLM5 and WRF v3.7.1) of the Irish climate that cover the period 1981–2016 and which have recently been completed and archived by researchers at ICHEC. These parameters represent the highest-resolution, hourly climate datasets that are currently available for Ireland for the period concerned. These new datasets contain parameters that are currently not available elsewhere: surface and subsurface runoff; air density at turbine heights; CAPE 3 km, Showalter index and surface lifted index; soil temperature and moisture content at four (WRF) and eight (COSMO-CLM) levels. Additional data that covers 2017 will soon be added to the datasets.
Preliminary analysis shows that for annual 2 m temperature and precipitation, there is good agreement between the ICHEC datasets and other available datasets (Met Éireann 1 km gridded observations and MÉRA). We have also presented uncertainty estimates (error, standard deviation and MAE) for some of the basic parameters (2 m temperature, 10 m winds, sea-level pressure and precipitation) and for several other lesser-used parameters: 10 cm soil temperature (COSMO-CLM and WRF); CAPE 3 km, Showalter index and surface lifted index (COSMO-CLM).
Both COSMO-CLM and WRF show similar hourly error and variance for 2 m temperature and precipitation, with WRF showing lower error and variance for both 10 m wind speeds and pressure. At daily time scales, WRF shows lower precipitation error during wetter seasons (autumn and winter) whilst the reverse is true during drier seasons. Also at daily time scales, WRF consistently shows lower 10 cm soil temperature error, both overall and for each season.
Additionally, a preliminary analysis of CAPE 3 km, Showalter index and surface lifted index using radiosonde data from two locations (Valentia and Castor Bay) show low overall error for each parameter: 2.31 and −0.68 J kg−1 (CAPE 3 km); −0.05 and 0.43 (Showalter index); 0.06 and 1.08 (surface lifted index).
The uncertainty estimates reported here for hourly 2 m temperature, hourly and daily precipitation and hourly 10 m winds are comparable to those found for MÉRA. However, those found for hourly pressure are considerably higher whilst an analysis of mean soil temperatures (albeit at 10 cm rather than 20 cm) shows a different seasonal pattern (COSMO-CLM and WRF consistently over-estimate) to that seen in Gleeson et al. (2017).
Ongoing (and future) work has been (will be) undertaken to provide a more complete analysis of uncertainty for the numerous climate parameters available at ICHEC, at various spatial and temporal scales, utilising station (including wind turbine) and satellite (particularly solar) observations. Additionally, appropriate skill scores such as (but not limited to) hit rate, false alarm rate, Hannsen–Kuiper skill score and equitable threat score are/will be calculated for each model parameter. This analysis will also be applied to the MÉRA dataset as each parameter becomes available. Ultimately, the aim is to provide researchers with a definitive gridded climate dataset for Ireland.
There is currently no publicly available method for access to the COSMO-CLM and WRF datasets. However, details on their future public release will appear on ICHEC's website (https://www.ichec.ie, last access: 1 April 2019) during 2019. Upon request, the authors can provide access to the parameters described in Sect. 3.1–3.6.
JF composed this article and carried out the analysis. PN carried out the COSMO and WRF simulations. RMG and CW provided guidance and sample scripts during the analysis.
The authors declare that they have no conflict of interest.
This article is part of the special issue “17th EMS Annual Meeting: European Conference for Applied Meteorology and Climatology 2017”. It is a result of the EMS Annual Meeting: European Conference for Applied Meteorology and Climatology 2017, Dublin, Ireland, 4–8 September 2017.
The authors would like to thank Emily Gleeson and Eoin Whelan for facilitating the download of the MÉRA data described in this article. We would also like to thank Seamus Walsh at Met Éireann for providing the gridded observation datasets. The research presented in this article was funded by the EPA Research Programme 2014–2020 Research Fellowship Grant 2016-CCRP-FS.28.
This paper was edited by Andrea K. Kaiser-Weiss and reviewed by Deborah Niermann, Per Unden, and one anonymous referee.
Bollmeyer, C., Keller, J. D., Ohlwein, C., Wahl, S., Crewell, S., Friederichs, P., Hense, A., Keune, J., Kneifel, S., Pscheidt, I., Redl, S., and Steinke, S.: Towards a high-resolution regional reanalysis for the European CORDEX domain, Q. J. Roy. Meteor. Soc., 141, 1–15, https://doi.org/10.1002/qj.2486, 2015.
Dasari, H. P. and Challa, V. S.: A study of precipitation climatology and its variability over Europe using an advanced regional model (WRF), American Journal of Climate Change, 4, 22–39, https://doi.org/10.4236/ajcc.2015.41003, 2015.
Dahlgreen, P., Landelius, T., Kallberg, P., and Gollvik, S.: A high-resolution regional reanalysis for Europe. Part 1: Three-dimensional reanalysis with the regional High-Resolution Limited-Area Model (HIRLAM), Q. J. Roy. Meteorol. Soc., 142, 2119–2131, https://doi.org/10.1002/qj.2807, 2016.
Dee, D. P., Uppala, S. M., Simmons, A. J., Berrisford, P., Poli, P., Kobayashi, S., Andrae, U., Balmaseda, M. A., Balsamo, G., Bauer, P., Bechtold, P., Beljaars, A. C. M., van de Berg, L., Bidlot, J., Bormann, N., Delsol, C., Dragani, R., Fuentes, M., Geer, A. J., Haimberger, L., Healy, S. B., Hersbach, H., Hólm, E. V., Isaksen, L., Kållberg, P., Köhler, M., Matricardi, M., McNally, A. P., Monge-Sanz, B. M., Morcrette, J.-J., Park, B.-K., Peubey, C., de Rosnay, P., Tavolato, C., Thépaut, J.-N., and Vitart, F.: The ERA-Interim reanalysis: configuration and performance of the data assimilation system, Q. J. Roy. Meteor. Soc., 137, 553–597, https://doi.org/10.1002/qj.828, 2011.
Di Luca, A., Argüeso, D., Evans, J. P., de Elía, R., and Laprise, R.: Quantifying the overall added value of dynamical downscaling and the contribution from different spatial scales, J. Geophys. Res.-Atmos., 121, 1575–1590, https://doi.org/10.1002/2015JD024009, 2016.
Feser, F., Rockel, B., von Storch, H., Winterfeldt, J., and Zahn, M.: Regional climate models add value to global model data: a review and selected examples, B. Am. Meteorol. Soc., 92, 1181–1192, 2011.
Gibson, J., Kallberg, P.W., Uppala, S., Hernandez, A., Nomura, A., and Serrano, E.: ERA 15, Re-Analysis (ERA) Project Report Series, ECMWF, 1997.
Gleeson, E., Whelan, E., and Hanley, J.: Met Éireann high resolution reanalysis for Ireland, Adv. Sci. Res., 14, 49–61, https://doi.org/10.5194/asr-14-49-2017, 2017.
Kanamitsu, M. and Kanamaru, H.: Fifty-seven-year reanalysis downscaling at 10 km (CaRD10). Part I: system detail and validation with observations, J. Climate, 20, 5553–5571, 2017.
Kendon, E., Roberts, N., Senior, C., and Roberts, M.: Realism of rainfall in a very high-resolution regional climate model, J. Climate, 25, 5791–5806, 2012.
Koo, M.-S., Choi, H.-J., and Han, J.-Y.: A Parameterization of Turbulent-Scale and Mesoscale Orographic Drag in a Global Atmospheric Model, J. Geophys. Res.-Atmos., 123, 8400–8417, 2018.
Lucas-Picher, P., Wulff-Nielsen, M., Christensen, J., Aðalgeirsdóttir, G., Mottram, R., and Simonsen, S. B.: Very high resolution regional climate model simulations over Greenland: Identifying added value, J. Geophys. Res., 117, D02108, https://doi.org/10.1029/2011JD016267, 2012.
Nolan, P., Goodman, P., O'Sullivan, P., Sweeney, C., Gleeson, E., and McGrath, R.: Climate change: impacts on Irish temperatures, in: Ireland's climate: the road ahead, edited by: Gleeson, E., McGrath, R., and Treanor, M., Met Éireann, Dublin, Ireland, 33–40, 2013a.
Nolan, P., McGrath, R., Gleeson, E., and Sweeney, C.: Impacts of climate change on Irish precipitation, in: Ireland's climate: the road ahead, edited by: Gleeson, E., McGrath, R., and Treanor, M., Met Éireann, Dublin, Ireland, 57–62, 2013b.
Nolan, P., O'Sullivan, J., and McGrath, R.: Impacts of climate change on mid-twenty-first century rainfall in Ireland: a high-resolution regional climate model ensemble approach, Int. J. Climatol., 37, 4347–4363, 2017, https://doi.org/10.1002/joc.5091, 2017.
Prein, A. F. and Gobiet, P.: Impacts of uncertainties in European gridded precipitation observations on regional climate analysis, Int. J. Climatol., 37, 305–327, 2017.
Rockel, B., Will, A., and Hense, A.: The regional climate model COSMO-CLM (CCLM), Meteorol. Z., 17, 347–348, 2008.
Schulzweida, U.: Climate Data Operators (CDO) User Guide Version 1.9.3, Max Planck Institute for Meteorologie, available at: https://code.mpimet.mpg.de/projects/cdo/embedded/cdo.pdf (last access: 1 April 2019), 2018.
Skamarock, W. C., Klemp, J. B., Dudhia, J., Gill, D. O., Barker, D. M., Duda, M. G., Huang, X. Y., Wang, W., and Powers, J. G.: A Description of the Advanced Research WRF Version 3, National Center for Atmospheric ResearchBoulder, Colorado, USA, NCAR Technical Note, NCAR/TN-475_STR, 2008.
Uppala, S. M., Kållberg, P. W., Simmons, A. J., Andrae, U., Da Costa Bechtold, V., Fiorino, M., Gibson, J. K., Haseler, J., Hernandez, A., Kelly, G. A., Li, X., Onogi, K., Saarinen, S., Sokka, N., Allan, R. P., Andersson, E., Arpe, K., Balmaseda, M. A., Beljaars, A. C. M., Berg, L. V. D., Bidlot, J., Bormann, N., Caires, S., Chevallier, F., Dethof, A., Dragosavac, M., Fisher, M., Fuentes, M., Hagemann, S., Hólm, E., Hoskins, B. J., Isaksen, L., Janssen, P. A. E. M., Jenne, R., Mcnally, A. P., Mahfouf, J.-F., Morcrette, J.-J., Rayner, N. A., Saunders, R. W., Simon, P., Sterl, A., Tren- berth, K. E., Untch, A., Vasiljevic, D., Viterbo, P., and Woollen, J.: The ERA-40 re-analysis, Q. J. Roy. Meteor. Soc., 131, 2961– 3012, https://doi.org/10.1256/qj.04.176, 2005.
Walsh, S.: A Summary of Climate Averages for Ireland 1981–2010, Met Éireann, Dublin, Climatological Note No. 14, 2012.
Walsh, S.: Long-term rainfall averages for Ireland, 1981–2010, Met Éireann, Dublin, Climatological Note No. 15, 2016.
Walsh, S.: Long-term temperature averages for Ireland, 1981–2010, Met Éireann, Dublin, Climatological Note No. 16, 2017.
Whelan, E., Hanley, J., and Gleeson, E.: 'The MÉRA Data Archive', Met Éireann, 2017-08-04, Met Éireann Technical Note, 65, 2017.